By Lina Sorg
Course-based undergraduate research experiences (CUREs) serve as unique opportunities to engage undergraduate students in research projects that extend well beyond the limitations of traditional mentoring. Topics in mathematical biology are especially appealing to a broad student demographic because of the accessible applications, which showcase the power of mathematics in the real world. During a minisymposium presentation at the 2024 SIAM Conference on the Life Sciences, which is currently taking place in Portland, Ore., Ph.D. student Haley Colgate Kottler of the University of Wisconsin-Madison discussed her experiences as a graduate student CURE mentor within the Madison Experimental Mathematics Lab (MXM). Founded in 2021, MXM supports undergraduate research in UW-Madison’s Department of Mathematics by allowing undergraduates, graduate students, and faculty to collaborate on mathematics-based projects and develop exploratory software.
In this program, teams of three to four undergraduates work with a mathematics faculty member and graduate student to complete a semester-long research project that is worth three credits. Professors propose project topics, recruit graduate mentors, and invite undergraduates to apply and rank their top choices. “Basically anything that includes some sort of interdisciplinary aspect and anything that mentions data gets a lot of applications,” Kottler said. The program is quite popular, as roughly 100 students—some of them repeat applicants from previous projects—compete for 40 spots. Accepted students must commit to 10 hours of work per week and meet regularly with both graduate and faculty mentors.
The first cohort of projects ran in spring 2022; since then, subjects have ranged from “Sports Analytics” and “Algebra in Systems Biology” to “An SIR Model of Adderall Use Disorder in College Students” and “Causality-based Data-driven Model Identification and Dynamical Interpolation.” Once students receive their assignments, the MXM CURE format follows a set of checkpoints:
- A kickoff meeting that establishes expectations for the coming semester
- A mid-semester check-in event, during which Group members share their progress in a five-minute presentation
- An end-of-semester open house and poster session
- A five-page final report that should be accessible to other mathematics students.
During course-based undergraduate research experiences within the Madison Experimental Mathematics Lab at the University of Wisconsin-Madison, three to four undergraduates, a graduate student, and a faculty mentor work together on semester-long mathematical research projects with real-world applications. Public domain image.
Kottler began by overviewing several recurring challenges across project domains. For instance, coordinating everyone’s schedule is routinely problematic. “Advanced undergrads have very busy schedules, and it’s hard to find time for everyone to meet,” Kottler said. “This has been a problem across the board.” To mitigate this issue, mentors and faculty are flexible with timing and occasionally meet with undergraduates in smaller subgroups. Kottler suggested that it might be more effective to have students sign up for projects in specific time blocks, much like how they register for classes.
In many cases, undergraduate students also struggle with prioritization. Most traditional coursework has clear to-do lists and consequences that are associated with a lack of delivery, but research is naturally quite different in that self-deadlines are more flexible. “Helping them prioritize can be pretty challenging,” Kottler said. “After every meeting, we had them create a task list of things they were trying to do by the following week.”
Next, Kottler reflected on the specific spring 2023 project for which she was a mentor: “Task Design to Distinguish Between Model-free and Model-based Reinforcement Learning.” Her team—which consisted of two men and two women who ranged from a sophomore to a graduating senior—sought to develop a task that is suitable for both human and computer use in order to better differentiate between model-free and model-based learning. Using mathematical frameworks from probability and machine learning, students developed a psychology task and simulated that task with code. While some of the students had extensive coding experience, none had any background in psychology.
Kottler quickly learned that one semester does not allow enough time for students to fully immerse themselves in the necessary background of a truly interdisciplinary project. After the mid-semester check-in revealed gaps in basic psychology knowledge, Kottler asked the undergraduates to read the original psychology paper that proposed the task, as well as an accessible machine learning book that offered some basic vocabulary. “An important part of mentoring is providing necessary logistical support, and recognizing that math may not be what the students need help with,” she said. Kottler also realized that many students do not equate math with groupwork and were not initially thinking about the task as a group project. Significant variability in coding expertise brought about additional challenges, as did the students’ inexperience with presenting mathematical research.
When the semester concluded, Kottler and her students continued the project in a less formal setting. The team met sporadically when they had time, restarted some of the code from scratch, simplified their algorithms, and finalized the simulations. Kottler also took a more active role in debugging and editing the actual code. “They didn’t really need help with the math,” she said. “It was more like, ‘how do I get this graph to look the way it should.’”
As a result of their hard work, three of the four undergraduates presented a poster on this topic at the 2024 Joint Mathematics Meetings earlier this year. The group also recently submitted a preprint based on their research [1], and two of the students are now pursuing advanced degrees in applied mathematics and management science and engineering.
References
[1] Li, P., Vineyard, J., Oh, S., Maloney, J., Cochran, A.L., & Kottler, H.C. (2024). Integrating local learning into the two-stage Markov task to separate model-based from model-free learning. Preprint, PsyArXiv.
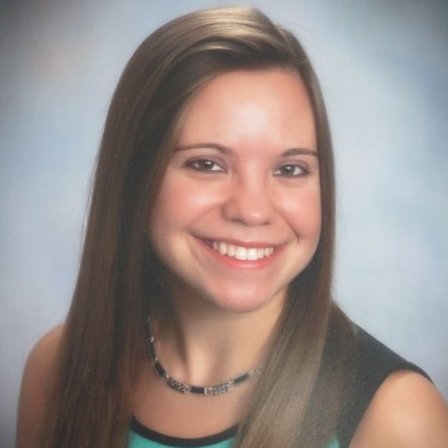
|
Lina Sorg is the managing editor of SIAM News. |