By Lakshmi Chandrasekaran
In the early 17th century, two French scientists successfully extracted quinine—a potent medication that is highly effective against malaria [2]—from the bark of the cinchona tree. Since then, physicians and researchers have raced to treat and prevent the disease. Chloroquine, a synthetic alternative of quinine that was produced to counter the drug’s scarcity during World War II, quickly became the medication of choice to combat malaria. Despite the prevalence of effective remedies, sub-Saharan Africa remains a malarial hotbed. The malaria parasite’s ongoing evolution towards drug resistance is a primary challenge in the fight against the epidemic.
The story of choloroquine resistance is particularly illuminating. Resistance to this drug first developed along the Thai-Cambodian border in the late 1950s [3], but malaria did not appear in Africa until 1978. Over a decade later, choloroquine resistance spread rapidly across the African continent with devastating consequences.
“The same happened with sulfadoxine-pyrimethamine, the next popular treatment for malaria,” Silvie Huijben, malaria expert and assistant professor at Arizona State University, said. “Now history appears to repeat itself for the current artemisinin-based drugs.” Resistance to these drugs has emerged and spread in Southeast Asia, and it is only a matter of time before it reaches Africa.
Why does a high-transmission setting like Africa experience delayed evolution of drug resistance? Mary Bushman of Emory University investigates this question using a set of mathematical models to track the dynamics of a population of humans, mosquitoes, and the parasites that circulate among them [1]. “We used a ‘nested’ model to simulate the infection dynamics within each individual host, and a stochastic transmission framework to allow transmission of parasites from humans to mosquitoes and vice versa,” Bushman said. A within-host model governs the population dynamics of the parasites once they are transmitted from a mosquito to a human.
Within-host and Between-host Dynamics
Parasites in the form of merozoites (an extracellular stage capable of initiating a new developmental cycle) infect red blood cells (RBCs) and grow within them, destroying the cells in the process. The RBC \((X)\) dynamics are modeled as
\[\frac{dX}{dt}=B-\alpha_XX-\beta X(S_1+S_2), \tag1 \]
where \(B\) is the production rate of new RBCs, \(\alpha_X\) is the death rate of uninfected RBCs, and \(\beta\) represents the rate of RBC infection by free merozoites.
The model explores the evolution of drug resistance by detailing the dynamics of RBCs infected with both drug-sensitive (S1) and drug-resistant (S2) parasites. The population of RBCs infected with either form of the parasite is denoted as Y1 or Y2. “Allowing the model to capture sensitive and resistant parasite dynamics in the same host yields realistic interactions that affect transmission as well as processes occurring at the epidemiological level,” Bushman said.
In the following equations, subscripts \(i\) and \(j\) denote the type-specific variables and parameters, i.e., \((i, j)= (1,2)\) or \((2, 1)\). An infected RBC of type \(i\) is modeled as
\[\frac{dY_i}{dt} = \beta XS_i - \frac{1}{1-e_i}\alpha_YY_i - \gamma Y_i - \delta_Z ZY_i - \delta_I (I_i + \omega_j I_j)Y_i. \tag2\]
The rate of merozoite \((S_i)\)-infected RBCs is countered by the death rate \((\alpha_Y)\) of infected RBCs and is further facilitated by the drug treatment (\(e_i = 0\) in the absence of antimalarial drugs and \(e_i = \varepsilon_i\) when the drug is present; \(\varepsilon_i\) represents the drug’s efficacy against type \(i\)). To account for the role of the body’s defense mechanism, Bushman models parasite-immune system interactions with elimination by both innate \((Z)\) and adaptive immunity \((I_i, I_j)\).
Innate immunity acts in a strain-independent manner that is critical to controlling parasitic growth during the acute phase of infection. On the other hand, adaptive (acquired) immunity is parasite-specific and required for infection clearance. Parasites trigger both immune responses, which decay in their absence. However, innate immunity turns on and off at a much higher rate than adaptive immunity, which takes longer to develop and decay.
Antigenic variation—a strategy that parasites have developed to “beat” acquired immunity—further complicates adaptive immunity dynamics by preventing the body’s adaptive immune response from recognizing parasites; this helps the latter survive. “We were the first group to model immunity in a quasi-mechanistic way that displays the right dynamics while still being tractable,” Bushman noted. “Past models that included immunity sort of ignored the within-host dynamics and assumed that one returns from infected to uninfected or recovered states independently of whatever is happening within the host.” But that does not occur biologically for any pathogen.
The infected RBCs produce more merozoites that can in turn infect new RBCs. The dynamics of free merozoites \((S)\) of type \(i\) are modeled as a combination of fitness costs \((\varphi_i)\) associated with drug resistance, natural merozoite death (death rate \(\alpha_S\)), and killing by innate \((Z)\) and adaptive immunity \((I_i, I_j)\):
\[\frac{dS_i}{dt} = R\alpha_Y(1-\varphi_i)Y_i - \alpha_SS_i - \beta XS_i - \delta_ZZS_i - \delta_I(I_i + w_j I_j)S_i. \tag3\]
To continue the next phase of the parasite’s life cycle in the mosquito, some infected RBCs give rise to gametocytes (cells that divide to form gametes capable of sexual reproduction) rather than merozoites. Since these are produced in very low amounts, they are unlikely to elicit an adaptive immune response.
The following equation describes gametocyte dynamics:
\[\frac{dG_i}{dt} = \gamma Y_i - \alpha_GG_i - \delta_ZZG_i, \tag4\]
with \(\gamma\) signifying birth rate. The remaining terms collectively represent gametocyte elimination, with \(\alpha_G\) (denoting natural death rate) and the last term representing killing by innate immunity.
The between-host model describes the feedback loop of human-to-mosquito interaction, which is modeled via stochastic principles, i.e., each human host is bitten by an assigned number of mosquitoes in a given day, drawn from a Poisson distribution with a specific mean.
Evolution of Drug-resistant Parasites in the Presence of Anti-malarial Drugs
Bushman and her colleagues first tested the model’s behavior in the absence of antimalarial drugs. “We wanted to determine what would happen if you have a population of completely drug-sensitive parasites and just throw a handful of drug-resistant infections in there without any drugs,” she said. As Figure 1 indicates, the resistant parasites—identified by the red curve—persisted in low-frequency, low-transmission settings for several years. In contrast, the resistant strains died down pretty quickly in high-transmission scenarios.
Figure 1. Introduction of drug-resistant parasites in low-transmission and high-transmission settings (left and right panels respectively) with no antimalarial drug use. Each panel summarizes 10 independent simulations with identical parameters and starting conditions; solid lines indicate mean values and shaded areas depict the range of observed values. The blue, red, and black coloring represents the number of hosts infected with drug-sensitive, drug-resistant, or both types of parasites (mixed infections). Figure courtesy of Mary Bushman.
What happens when the drug is introduced? As in the previous case, Figure 2 demonstrates that the drug-resistant strain persists in the low-transmission setting. However, the resistant strain does not die out in the high-transmission situation, but rather establishes itself over time and actually spreads faster than in low-transmission circumstances. How does this happen?
Figure 2. Introduction of drug-resistant parasites in low-transmission and high-transmission settings (left and right panels respectively) with ongoing antimalarial drug use. Each panel summarizes three independent simulations with identical parameters and starting conditions; solid lines indicate mean values and shaded areas depict the range of observed values. The blue, red, and black coloring represents the number of hosts infected with drug-sensitive, drug-resistant, or both types of parasites (mixed infections). Figure courtesy of Mary Bushman.
Bushman reasons that a high-transmission setting presents a challenging environment for the resistant parasite’s survival, as most hosts harbor the drug-sensitive parasite; the resistant one thus falls victim to within-host competition. This complicates a resistant strain’s effort to establish itself in a high-transmission setting.
Bushman compares the high and low transmission settings to an overcrowded versus sparse garden. “If you plant some seeds or resistant strains in a crowded garden already teeming with different kinds of plants, most of the new seeds won’t take root due to lack of space,” she said. “But if the garden is sensitive to a herbicide and you throw in a herbicide-resistant flower, the latter would fill up the entire garden as the herbicide would have killed everything else.” This analogy explains why a resistant parasite spreads quickly after overcoming the initial barrier and establishing itself. Drug treatments in high-transmission areas paradoxically lead to the establishment and spread of drug resistance.
These findings emphasize the fundamental differences regarding the evolution of drug resistance in various settings. Bushman’s model explains both the delayed appearance of chloroquine resistance in Africa and its rapid spread upon emergence. “It has not previously been recognized that the high prevalence of infection might, in itself, provide a sort of protection against the evolution of drug resistance,” she observed.
The results from Bushman’s study will be instructive for policymakers and healthcare professionals who are planning or implementing malaria control measures, particularly in high-transmission settings. “One of the most important deductions from this work is that intense malaria control and eradication activities aimed at reducing transmission could lead to a higher risk of antimalarial resistance evolution in sub-Saharan Africa,” Huijben said.
Bushman concluded with her own caveat to this sentiment. “[This is not to say that] we shouldn’t aim to reduce malaria burden,” she said. “But we should be mindful of the opportunity such reductions provide for drug resistances to emerge, and plan accordingly.”
References
[1] Bushman, M., Antia, R., Udhayakumar, V., & de Roode, J.C. (2018). Within-host competition can delay evolution of drug resistance in malaria. PLOS Biol., 16(8), e2005712.
[2] Cassauwers, T. (2015, December 30). The global history of quinine, the world’s first anti-malaria drug. Medium. Retrieved from https://medium.com/@tcassauwers/the-global-history-of-the-world-s-first-anti-malaria-drug-d1e11f0ba729.
[3] Flegg, J.A., Metcalf, C.J.E., Gharbi, M., Venkatesan, M., Shewchuk, T., Sibley, C.H., & Guerin, P.J. (2013). Trends in Antimalarial drug use in Africa. Amer. J. Trop. Med. Hyg., 89(5), 857-865.
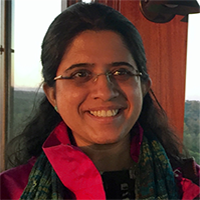 |
Lakshmi Chandrasekaran received her Ph.D. in mathematical sciences from the New Jersey Institute of Technology. She earned her masters in science journalism from Northwestern University and is a freelance science writer whose work has appeared in several outlets. She can be reached on Twitter at @science_eye. |