By Achyut Tiwari
The concept of risk and its relevant applications is undoubtedly complex. One recent example is the COVID-19 pandemic, during which decision-makers around the world faced uncertainty about when to employ strict lockdowns and enforce restrictions like mask mandates, travel limitations, and so forth. Interestingly, Sweden fared relatively well during the pandemic when compared to other countries — despite the fact that it did not impose any major mandatory lockdowns [3].
In retrospect, it is easy to pass judgment on governmental reactions to the pandemic, including policies that resulted in significant economic losses [2]. However, current commentators have the benefit of hindsight. At COVID-19’s onset, decision-makers possessed minimal to no knowledge about the virus and its potential impacts; as such, most governing bodies erred on the side of caution.
Figure 1. Visual depiction of the Lindy effect. Figure courtesy of [4].
Science and mathematics can help society process the world’s random nature, understand potential aftereffects, and react accordingly. When facing the unknown and making critical decisions that will impact future generations, authorities must identify the framework that seems most suitable. This is where the Lindy effect—a framework that makes sense of the “test of time” as a concept—comes into play (see Figure 1).
Mathematically, we can express the relation that is postulated by the Lindy effect with the following statement about a random variable \(T\), which corresponds to the lifetime of an object and is assumed to take values in the range \(c \le T < \infty\) (with a lower bound \(c \ge 0\)) [1]:
\[E [T-t \mid T > t]= p \cdot t.\]
The left side of the formula denotes the conditional expectation of the remaining lifetime \(T-t\), given that \(T\) has exceeded \(t\). The parameter \(p\) on the right side—which Iddo Eliazar calls the “Lindy proportion”—is a positive constant [1].
This formulation is equivalent to setting the survival function of \(T\) as
\[\Phi(t): = \Pr (T>t)=\Big(\frac{C}{t}\Big)^\epsilon, \textrm{ where } \epsilon=1+ \frac{1}{p},\]
which has the hazard function
\[-\frac{\Phi'(t)}{\Phi(t)}=\frac{\epsilon}{t}=\frac{1+p}{p}\frac{1}{t}.\]
The lifetime \(T\) thus follows a Pareto distribution (a type of power-law distribution) with exponent \(\epsilon\).
Conversely, only Pareto distributions with exponent \(1<\epsilon<\infty\) correspond to a lifetime distribution that satisfies the Lindy effect, since the Lindy proportion \(p\) must be positive and finite (in particular, we assume that the lifetime \(T\) has a finite expectation value) [1]. Eliazar proposed an alternative formulation of the Lindy effect that involves the median instead of the mean of the remaining lifetime \(T-t\), which pertains to Pareto distributions for the lifetime \(T\) with the full range of possible Pareto exponents \(0 <\epsilon < \infty\). Simply put, the Lindy effect is the idea that the older something is, the longer it is likely to persist in the future.
Due to the rise of relatively cheap computational power and novel machine learning applications, recent years have seen many predictions around a wide variety of dynamic problems. The most misleading output of these projects is the purported determinacy of their conclusions. Studying a problem in a static manner with very limited data and drawing conclusions as absolute truth is what I call statistical hallucination; in reality, these conclusions are mere conjecture.
Ultimately, navigating the unpredictable nature of the world requires a pragmatic approach. While statistical conjectures do help researchers deepen their overall understanding of specific problems, it is difficult to build a strong case regarding their definiteness. The Lindy effect thus emerges as a robust guide for decision-makers who face uncertainty when making decisions that affect the wellbeing of society in the long term — especially in the face of statistical conjectures and the evolving landscape of technology. By embracing the enduring wisdom of the Lindy effect, we ensure a more resilient path forward in the complex tapestry of the unknown.
The views in this article are personal to the author.
References
[1] Eliazar, I. (2017). Lindy’s law. Physica A: Stat. Mech. Appl., 486, 797-805.
[2] Lewis, D. (2022). What scientists have learnt from COVID lockdowns. Nature, 609(7926), 236-239.
[3] Ludvigsson, J.F. (2022). How Sweden approached the COVID‐19 pandemic: Summary and commentary on the National Commission Inquiry. Acta Paediatr., 112(1), 19-33.
[4] Pedro, S. (2020, May 20). What is the Lindy effect? Medium. Retrieved from https://medium.com/@samuelpedro1992/what-is-the-lindy-effect-3c1d6ec96a08.
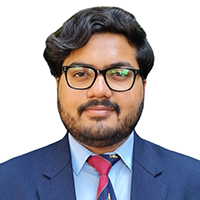 |
Achyut Tiwari is a programmer and writer who serves as a data engineer at Tata Consultancy Services in India. He is the founding president of the Jaypee University of Information Technology SIAM Student Chapter. Tiwari’s research combines machine learning and dynamical systems to apply computational methodologies in bioinformatics and other fields of engineering. He is a member of SIAM and the Association for Computing Machinery. |