By Lina Sorg
Convective flow in the Earth’s fluid outer core, collectively called the geodynamo, generates and maintains the Earth’s intrinsic and time-varying magnetic field. This dipole field changes on a variety of different timescales, which vary from decades to millions of years, and can even completely reverse its orientation over very long periods. However, Kyle Gwirtz of the University of Arizona is interested in shorter timescales. He hopes to forecast changes more effectively by understanding the dynamics in the outer core that drive these changes. During a virtual minisymposium presentation at the 2020 SIAM Conference on Mathematics of Planet Earth, which took place earlier this month, Gwirtz spoke about his efforts to create a reduced-order model of the geodynamo that assesses data assimilation (DA) systems for decadal-scale geomagnetic forecasting.
Earth-like, three-dimensional (3D) numerical models of the geodynamo system have existed for roughly 25 years. These representations fully model fluid motion in the Earth’s core, which is coupled to the geomagnetic field. Information about the magnetic field stems from a variety of sources, including satellite observations, paleomagnetic reconstructions, and terrestrial-based surveillance. Ship sailing logs, magnetic material in ancient pottery and bricks, and the configuration of cooled rocks also supply relevant data. In response, researchers have begun using DA techniques to combine current numerical models with observations about the geomagnetic field.
The goal of Gwirtz’s study is to understand the impact of DA techniques on decadal-scale geomagnetic forecasting. However, DA in the context of geomagnetism presents unique challenges. Several complications are immediately apparent, including the fact that the geodynamo is both chaotic and nonlinear. Detailed, high-resolution simulations are also computationally expensive, which limits the ensemble size. And running a high-dimensional system with a very small ensemble yields problems with the associated statistics. Numerical geodynamo models also operate in a parameter regime that is unlike Earth; this keeps things computationally tractable but means that some systematic errors exist in the forecast. Furthermore, the exact relation between model time and geophysical time is unknown. Therefore, scientists must find a way to rescale time as they select parameter regimes that yield a dynamo that somewhat resembles Earth. Unfortunately, less-than-optimal rescaling efforts can lead to mistimed observations.
In addition, only observations of the magnetic field are available for assimilation; the velocity field goes unobserved, which further complicates the situation. And finally, global observations appear in the form of spherical harmonic coefficients, meaning that physical intuition for certain DA techniques may not be applicable. These last two difficulties are the most significant.
Gwirtz attempts to approach this complex problem with a reduced-scale model, given that progress in DA and other sciences is partly attributable to the availability of simplified models. He creates a “wish list” for the exploration of geomagnetic DA. As will all models of the geodynamo, a useful reduced-order model in this context must be chaotic and nonlinear. The model must also be complex enough to provide useful insight while simultaneously remaining relatively computationally inexpensive. “We want a level of complexity that’s interesting, but we want the model to be computationally inexpensive enough that we can run a lot of experiments,” Gwirtz said.
Because numerical geodynamo models operate in a parameter regime that is different from Earth, model bias is also important. “We need something with adjustable parameters so we can simulate this issue of having systematic model error from incorrect parameter values,” Gwirtz said. Lastly, a simplified model must also be amenable to observations in spectral space due the makeup of the geodynamo (which comprises an observed magnetic field that is coupled to the outer core’s unobserved velocity field).
Gwirtz recently finalized the setup of his reduced-order model of the geodynamo on the surface of a sphere.
Gwirtz and his team decide on a simple magnetohydrodynamic (MHD) system because it effectively represents a dynamo. This type of system consists of a velocity field coupled to an induction equation. Because they wish to run a large number of numerical experiments, they restrict themselves to two dimensions and avoid solving the Navier-Stokes equation to produce a chaotic velocity field; doing so is too computationally expensive. Instead, they turn to the two-dimensional (2D) Kuramoto-Sivashinsky (KS) equation, which is simple, fast, chaotic, and computationally inexpensive. The solution to this equation yields a scalar field, and Gwirtz and his collaborators treat this field as if it reflects the vorticity component that is perpendicular to the 2D domain. They thus recover the underlying velocity field by assuming that it is divergence-free.
Next, the researchers modify the KS equation by adding a term to reflect the influence of the Lorentz force in an MHD system. Because they are building a magneto-convection system rather than a self-sustaining dynamo, they assume that the total magnetic field is a sum of the constant background field and a time-varying perturbation. They also presume that the perturbation is described by a vector potential that is perpendicular to the 2D domain. In short, they describe the magnetic field with just a 2D scalar field. The complete model ultimately consists of the modified KS equation that is coupled to the induction equation.
Upon completion of their model, Gwirtz and his team conduct several experiments—including synthetic data experiments with the model in the cartesian plane—to observe the magnetic field and its associated spectral information. The ensemble Kalmar filter (EnKF), which Gwirtz describes as a “flavor” of DA, demonstrates the ability to reduce the amount of error and accurately estimate the underlying velocity field in this setting, which enables the creation of forecasts. They are particularly interested in maintaining a small ensemble size because of the computational expense, and thus identify optimal approaches that are in spirit like localization and allow them to achieve the same results with a limited ensemble.
Finally, Gwirtz and his colleagues have recently finalized the setup of their reduced-order model on the surface of a sphere, which allows for spectral observations in the form of spherical harmonics. This more closely reflects the true situation in geomagnetic DA, though some complications arise from the process of moving the model from a cartesian grid to a sphere’s 2D surface. Nevertheless, Gwirtz continues to explore schemes for the optimization of DA in this geometry and plans to subject any future efforts to his team’s suite of numerical experiments.
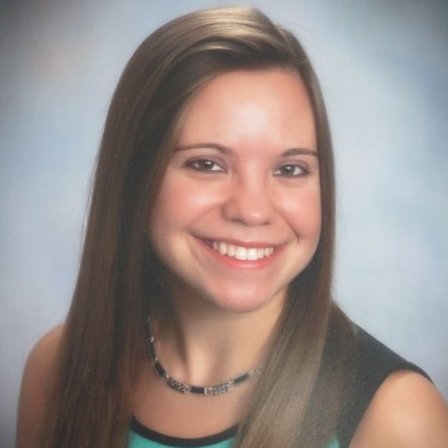 |
Lina Sorg is the managing editor of SIAM News. |