By Veronica Ciocanel, Nicholas Goldrosen, and Chad M. Topaz
Every year, tens of thousands of cases are judged in the U.S. federal criminal justice system, which comprises 94 district courts—at least one per state—and nearly 700 judges. To ensure that offenders are judged fairly, the U.S. government adopted federal sentencing guidelines in 1984 as part of the Sentencing Reform Act. However, the 2005 Supreme Court case United States v. Booker later made these guidelines only advisory. According to this decision, federal district court judges must still calculate the sentence that is recommended by the guidelines but can ultimately issue a different sentence if they deem it appropriate. As a result, critics of the 2005 verdict believe that the unequal treatment of cases causes significant variations in sentencing outcomes. Sentencing disparity in the federal courts is thus an active area of legal scholarship research.
The lack of complete data on federal sentencing limits the study of such disparities. The public does not have access to a comprehensive, high-quality dataset about federal criminal cases in the U.S., and while anyone can choose to attend a court proceeding, it is infeasible to do so on a large scale. However, some partial information is available. The U.S. Sentencing Commission (USSC) Datafiles provide detailed information about criminal cases and sentences, the Federal Judicial Center Integrated Database connects cases to court docket numbers, the Public Access to Court Electronic Records service gives the initials of the sentencing judge, and the Federal Judicial Center Biographical Directory of Article III Federal Judges houses detailed information about current judges. Previous work that sought to combine some of these databases and analyze sentencing disparities had to use data from proprietary sources, which prevented the disclosure of certain information like the identities of individual judges [4].
In our first contribution to the field, we aimed to compile a dataset that collects all publicly-available information about federal criminal sentencing decisions. Our resulting database is called JUSTFAIR (Judicial System Transparency through Federal Archive Inferred Records) and includes more than 600,000 records from 2001 to 2019, with information about defendants and their demographics, crimes and sentences, and the sentencing judges [3]. We validated JUSTFAIR—which employs a transparent data cleaning, processing, and consolidation pipeline—with data from available judicial opinions. Despite our efforts, the database is incomplete because data from certain sources or states were recorded in ambiguous ways that prevented us from matching the records. Nevertheless, JUSTFAIR is the first large-scale, free, and public resource on federal sentences — we hope that it provides a useful tool for educators, researchers, and other interested parties.
Many prior studies that used inferred records to examine variation in sentencing outcomes have assumed the random assignment of cases to judges within a district [1, 2, 4]. In theory, case distribution to judges is based on a random drawing to ensure that the net observable and unobservable characteristics of cases and defendants are similar across judges in a district. Previous smaller-scale studies of sentencing equity utilized statistical analysis (such as an F-test) [4] or Monte Carlo simulations [1] to verify the random assignment of cases. Interestingly, similar tests for JUSTFAIR revealed evidence of nonrandom assignment in most districts [7].
In an analysis of roughly 380,000 criminal sentences from 2006 to 2019, we found that the average defendant of any race receives a downward deviation from the minimum sentence that is specified in the guidelines. However, this deviation is larger for white defendants than for Black or Hispanic defendants. Case and defendant variables—such as the guideline or statutory minimum sentence—account for a large portion of this disparity, but not all; when compared to white defendants, Black defendants receive 14 percent greater sentences and Hispanic defendants receive 10 percent greater sentences. This finding broadly agrees with other research [8, 11]. Of course, interpreting a residual difference in sentences (after controls) as disparity can be problematic. For instance, we cannot observe every defendant characteristic, as we do not have access to a detailed description of the offense conduct or the defendant’s interactions with the court at sentencing. Racial bias might also manifest when determining many of these variables, such as when deciding whether to add certain enhancements to increase the range of the guidelines. But given the apparent lack of random assignment, we are unable to assume that these controls are randomly distributed across judges.
Figure 1. Variation in percentage racial disparities by judge. Figure courtesy of Nicholas Goldrosen based on results from [7].
Since JUSTFAIR’s major contribution to sentencing research consists of linking cases to judges, we next analyzed sentence variations within each judge’s caseload. To do so, we fit a hierarchical linear model that nests defendants within judges, and estimated random slopes that reflect each judge’s conditional disparity between white and Black or white and Hispanic defendants’ sentences. The mean within-judge conditional disparity is 13 percent between white and Black defendants and 19 percent between white and Hispanic defendants. These within-judge disparities also vary greatly across judges themselves: a judge who is one standard deviation above average—14.9 percent of judges in our dataset for Black-white disparity and 15.2 percent for Hispanic-white disparity—has a 39 percent Black-white disparity or 49 percent Hispanic-white disparity (see Figure 1).
According to the 2019 and 2020 Annual Report and Sourcebook of Federal Sentencing Statistics, USSC research has also identified significant variation in sentencing across districts and judges. While this research has not examined racial disparity, the reports show that the individual judge greatly impacts the imposed sentence. In fact, judges within the same courthouse can widely range in their deviations from the applicable guideline minimum. Overall variation and racial disparity have increased under Booker’s 2005 advisory guidelines regime [10, 11]. Despite the fact that U.S. sentencing law instructs judges to “avoid unwarranted sentence disparities” amongst similarly situated defendants (18 USC §3553(a)(6)), there is a concerning variation between judges and based on defendant race. Of course, this judicial discretion has its own tradeoffs, as the mandatory guideline regime prior to 2005 reduced disparity but increased overall harshness [6]. Our results cannot in any way determine the “right” sentence for any given offense or defendant, but they do highlight the difficulty of calibrating the level of discretion that judges should possess.
The JUSTFAIR dataset is undoubtedly complex, but raw USSC data is even more so. At the moment, USSC provides one data file for each fiscal year from 2002 through 2021. Each file contains tens of thousands of records—ranging from around 60,000 to nearly 100,000—and each year has a slightly different set of variables that sometimes number in the tens of thousands. We found approximately 2,400 variables that are common across all years. Exploratory analysis of this data would be a valuable first step prior to statistical modeling, machine learning, and other quantitative approaches, but traditional methods of data exploration are ill-suited for this high-dimensional data. In the legal and criminological literature, a common strategy involves looking at pairwise interactions of variables (accounting for whether the two variables are numerical, categorical, or one of each). Because there are 2.9 million possible pairings among the 2,400 variables, one cannot practically follow this traditional approach to completion; even determining which pairings are worth examining is infeasible. When considering the relationship between three variables rather than two, the 2,400 variables give rise to 2.3 billion possible combinations.
In the face of such challenges, one can turn to well-known tools such as principal component analysis (PCA), multiple correspondence analysis, and factor analysis of mixed data. These dimensionality reduction techniques attempt to exploit associations between variables so that the data can be described more simply. Despite widespread use in the natural and social sciences, dimensionality reduction is rarely applied to criminal justice research. Regardless, even powerful techniques like PCA have limitations. For example, these techniques rely on correlations and are therefore ineffective when the data has nonlinear structure. Dimension reduction is an active area of research in statistics, applied mathematics, and data science that has given rise to newer techniques including Isomap and kernel PCA [5, 9]. We believe that these new functionalities will facilitate great opportunities for applied mathematicians to apply rigorous techniques to federal court datasets, in close collaboration with legal scholars and criminologists.
Veronica Ciocanel delivered a minisymposium presentation on this research at the 2022 SIAM Annual Meeting, which took place in Pittsburgh, Pa., last year.
References
[1] Abrams, D.S., Bertrand, M., & Mullainathan, S. (2012). Do judges vary in their treatment of race? J. Legal Stud., 41(2), 347-383.
[2] Anderson, J.M., Kling, J.R., & Stith, K. (1999). Measuring interjudge sentencing disparity: Before and after the federal sentencing guidelines. J. Law Econ., 42(S1), 271-308.
[3] Ciocanel, M.-V., Topaz, C.M., Santorella, R., Sen, S., Smith, C.M., & Hufstetler, A. (2020). JUSTFAIR: Judicial System Transparency through Federal Archive Inferred Records. PLoS ONE, 15(10), e0241381.
[4] Cohen, A., & Yang, C.S. (2019). Judicial politics and sentencing decisions. Am. Econ. J. Econ. Policy, 11(1), 160-91.
[5] Cunningham, J.P., & Ghahramani, Z. (2016). Linear dimensionality reduction: Survey, insights, and generalizations. J. Mach. Learn. Res., 16, 2859-2900.
[6] Fischman, J.B., & Schanzenbach, M.M. (2012). Racial disparities under the federal sentencing guidelines: The role of judicial discretion and mandatory minimums. J Empir. Legal Stud., 9(4), 729-764.
[7] Goldrosen, N., Smith, C.M., Ciocanel, M.-V., Santorella, R., Sen, S., Bushway, S., & Topaz, C.M. (2023). Racial disparities in criminal sentencing vary considerably across federal judges. J. Institutional Theor. Econ., 179(1), 92-113.
[8] Rehavi, M.M., & Starr, S.B. (2014). Racial disparity in federal criminal sentences. J. Polit. Econ., 122(6), 1320-1354.
[9] Sarveniazi, A. (2014). An actual survey of dimensionality reduction. Am. J. Comput. Math., 4(2), 55-72.
[10] Yang, C.S. (2014). Have interjudge sentencing disparities increased in an advisory guidelines regime? Evidence from Booker. NYU Law Rev., 89(4), 1268-1342.
[11] Yang, C.S. (2015). Free at last? Judicial discretion and racial disparities in federal sentencing. J. Legal. Stud., 44(1), 75-111.
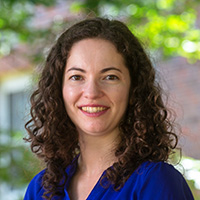 |
Veronica Ciocanel is an applied mathematician and mathematical biologist in the Departments of Mathematics and Biology at Duke University. She is also a member of the Institute for the Quantitative Study of Inclusion, Diversity, and Equity (QSIDE). |
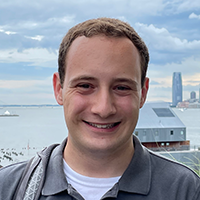 |
Nicholas Goldrosen is a Ph.D. student at the University of Cambridge’s Institute of Criminology. His research interests include policing and police misconduct, sentencing, and drug enforcement. |
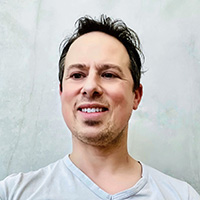 |
Chad M. Topaz is the co-founder of QSIDE, a professor of complex systems at Williams College, and an adjunct professor of applied mathematics (by courtesy) at the University of Colorado-Boulder. |