By Lina Sorg
Sickle cell disease (SCD) refers to a group of genetic red blood cell disorders wherein an abnormal type of hemoglobin causes the cells to become hard, sticky, and sickle shaped (see Figure 1). These sickled cells die prematurely, lose their flexibility, and occlude blood vessels, preventing blood flow throughout the body and inhibiting oxygen delivery to organs and tissues. As a result, individuals with SCD frequently experience pain crises and other health complications like infection, stroke, anemia, kidney problems, and acute chest syndrome.
Discomfort levels, symptoms and complications, and subsequent pain management strategies differ between pediatric versus adult SCD patients. Adults often face chronic, long-lasting pain as a result of gradual damage to blood vessels, which establishes a baseline level of pain. This baseline is not yet present in young patients. “With pediatric patients, the pain events are often acute and sporadic,” Reginald McGee of College of the Holy Cross said. “You’re going from zero to high pain fairly rapidly.” A distinct approach is therefore necessary to measure the acute episodic nature of pain events in children. During the
10th International Congress on Industrial and Applied Mathematics, which took place last week in Tokyo, Japan, McGee presented preliminary work that explores the interaction between sleep quality and pain levels in pediatric patients with SCD.
McGee relied on data from a statistical study entitled “Using E-Diaries to Assess Sleep and Pain in Children with Sickle Cell Disease,” for which his colleague Cecelia Valrie of Virginia Commonwealth University was the principal investigator. From 2010 to 2015, the study amassed data from 88 pediatric SCD patients between the ages of eight and 17. Participants completed ecological momentary assessments twice a day that detailed their pain levels, sleep quality, and other related aspects; each patient completed approximately 51 entries and reported pain nearly 20 percent of the time. Concurrent sleep actigraphy measurements—a noninvasive technique that uses an actimetry sensor to assess cycles of rest and activity—supplemented this self-reported information. The resulting dataset included a myriad of factors, such as demographics, SCD genotype, pain medications, wake behaviors (i.e., daytime tiredness and daytime activity levels), and affective components like stress.
Figure 2. When adults with chronic pain use drugs to manage their pain, drug dynamics spike and bounce around while gradually decaying down. Once drug uses ceases, pain levels eventually return to the nonzero steady state. Figure courtesy of Reginald McGee.
McGee combined this data with an existing model for chronic pain dynamics in adults [1]. A linear model of the patient’s demographic and medication information determines the background pain \(P_b\), and separate equations account for long-acting opioids, short-acting opioids, and non-opioid drugs. When individuals use drugs to manage their pain, drug dynamics spike and then bounce around while gradually decaying down (see Figure 2). Once drug uses ceases (around the 18-hour mark in Figure 2), pain levels eventually return to the nonzero steady state \(P_b\). However, this presents a challenge for the purposes of McGee’s study; because pediatric pain is acute, the pediatric steady state for SCD should be zero. As such, McGee adopted the existing model [1] to create a completely new pediatric model for SCD.
To do so, he focused specifically on the relationship between pain and sleep quality. The baseline pain number in the original model [1] stems from linear regression, but McGee instead sought to make baseline pain a function of sleep quality. “We want it to be proportional to the deviation from average sleep,” he said. Any time sleep quality drops below a certain threshold, the model will spike. But despite the new model firing when it should, it does not quantitatively fit the data very well. McGee hypothesized that repeated nights with sleep quality under the threshold might be causing the discrepancy. “It’s not just one bad night of sleep that’s going to get you,” he said. “It’s usually a couple of nights. As it begins to accumulate, that sleep debt starts to throw us off our game.”
So, McGee incorporated an accumulation of bad sleep into the model so that it fires when the average sleep quality for three consecutive days falls below the threshold. Though the model still does not quite exhibit a good quantitative fit with the data, it performs better than before. “As we spend more time with this patient-reported data, we’re having to reframe what we consider ‘good,’” McGee said, explaining that he and his colleagues are currently verifying whether the model results appropriately fall between reported values for each pain window and correctly identify the presence of pain. “Instead of looking at a quantitative fit, we’re trying to reframe in terms of prediction.” When viewed in this way, the model is more successful.
To further mitigate the issue with quantitative fit, McGee intends to incorporate additional data beyond sleep quality, identify the data components that best fit the model, and build a framework that will accept information from different subsets of the dataset — which might involve combining sleep quality with stress or other affective factors. He also wants to investigate the definitions of pain onset (any increase of \(P\) from \(0\)) and pain exacerbation (\(\Delta P >10\)) and reevaluate if necessary. “Which instances of the model framework perform best at classifying pain episode events?” McGee asked. He and his team look forward to exploring this and other questions.
References
[1] Clifton, S.M., Kang, C., Li, J.J., Long, Q., Shah, N., & Abrams, D.M. (2017). Hybrid statistical and mechanistic mathematical model guides mobile health intervention for chronic pain. J. Comput. Biol., 24(7), 675-688.
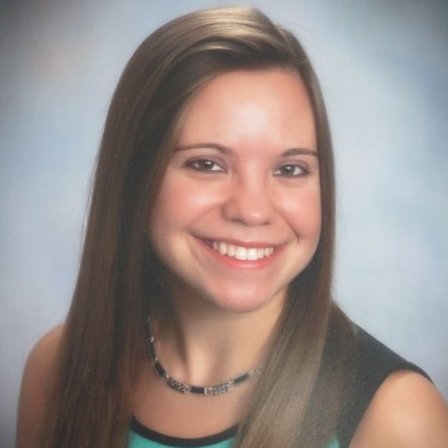
|
Lina Sorg is the managing editor of SIAM News. |