By Lina Sorg
As the capital city of Columbia, Bogotá has made major strides to decrease its crime rate and improve its reputation in recent years. Yet as a large metropolis and the most populated municipality in the country—with 7 million inhabitants—violent crimes and other misdemeanors are all but unavoidable. Nearly 3,000 surveillance cameras are currently operational in Bogotá. A camera fleet this large cannot possibly be monitored solely by humans. However, the mobile nature and unideal placement of the cameras means that artificial intelligence tools are also unable to handle the situation in its entirety. Thus, optimal surveillance likely requires a combination of AI techniques and human observation of the video feed. Bogotá plans to deploy 600 new security cameras in the coming year; determining which locations constitute ideal placement is therefore a critical task that requires computational modeling.
At ICIAM 2019, Alvaro Riascos described a predictive model which helps achieve optimal surveillance by law enforcement agencies in Bogotá, Columbia.
During a scientific session at the 9th International Congress on Industrial and Applied Mathematics, currently taking place in Valencia, Spain, Alvaro Riascos of the University of Los Andes described an existing predictive model used to efficiently allocate the location of surveillance cameras, police stations, and other law enforcement resources throughout the city. In 2012 and 2015, all murders and 25 percent of reported crimes in Bogotá took place in just two percent of street segments. Yet less than 10 percent of overall police patrolling time was dedicated to these same segments. “Are we simply ignoring the places where crime is happening?” Riascos asked. “Or are we just not able to pin down exactly where things are going to occur and deploy police accordingly?” These statistics motivated him to work with Bogotá, especially since policymakers must efficiently allocate scarce law enforcement resources with a vast and highly-dynamic environment.
Police stations in Bogotá are small, with simple allocation processes and only four to six policemen per shift. Because resources are so limited, it is important to ensure that the stations and patrol units are optimally located based on the time it takes the policemen to reach various points around the city. Of course, deploying police to different areas does not necessarily mean that crime rates will decrease, since criminals can always relocate their efforts to other locations. But at the very least, it provides insight into the dynamics of law enforcement.
After experimenting with various basic models and simple techniques, Riascos decided that the model in Mohler et al.’s 2011 paper—entitled “Self exciting point process modeling of crime”—had the greatest predictive power among a family of crime prediction models applied to real data from Bogotá. This model—which includes the crime itself, as well as the time and location of the incident—also marked the starting point of a three-year research agenda on crime prediction in Bogotá, financed by the Columbian government. It also inspired the authors to create a company that deploys policing software in over 50 cities worldwide, including New York, Los Angeles, and Chicago. Riascos implemented Mohler et al.’s crime prediction model in Bogotá.
Since police stations in Bogotá are small, it is important to ensure optimal location of stations and patrol units around the city.
Mohler et al.’s model is constructed under the following three assumptions, which allow Riascos to explore clustering of crime in time:
• Criminality concentrates in specific areas of the city
• There is a higher incidence of crime at certain times of day and certain days of the week
• Crime spreads from place to place and repeats itself as a seismic event.
The last assumption is perhaps the most interesting, as Mohler et al.’s model is motivated by an earthquake model that measures aftershocks. “There’s a lot of evidence that earthquakes and crimes take place like that,” Riascos said. “When some crime happens, it tends to happen close and repeatedly after the first crime occurs.”
He used these three assumptions to estimate an intensity function based on stochastic declustering techniques and kernel density estimation with variable bandwidth. Then he adapted the data for existing police activity. Policemen in Bogotá work for eight hours a day, so Riascos divided the workday into eight hours with the intent of directing police behavior during those hours. He also separated weekday and weekend behavior and treated the two slightly differently.
Riascos is only able to pin down two big hotspots for each of Bogotá’s 19 localities. “We can only identify 38 hotspots for the police at every single shift they have,” he said. “It’s definitely a question of allocating scare resources.” Riascos found that on average, Mohler et al.’s model captures 3.11 percent of crimes for each shift. This translates to a predictive accuracy index (PAI) of 22.29 — bearing in mind that the 38 hotspots of each shift cover only 0.14 percent of Bogotá’s total area. “For our standards, this is pretty good,” he said, adding that other models yielded PAIs no larger than 12.
Riascos’ application of an existing predictive model to the city of Bogotá allowed him to investigate current police activity and better allocate limited law enforcement resources. His identification of critical hotspots can help the Columbian government optimally place new surveillance cameras and police stations, deploy police units to critical areas, and ultimately reduce crime rates in the city.
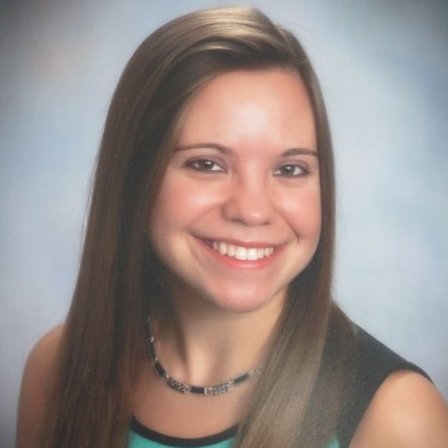 |
Lina Sorg is the associate editor of SIAM News. |