By Jayanth Kumar Narayana, Sanjay H. Chotirmall, and Krasimira Tsaneva-Atanasova
The human microbiome is a prime example of a complex system that is characterized by a rich, interacting, and multispecies community. Its significant involvement in health and disease—including chronic lung disease—is widely recognized. Indeed, an unbalanced respiratory microbial ecosystem can provoke a range of negative effects on host biology. One example is an uncontrolled immune response, which in turn affects the microbial environment in patient airways, promotes further disbalance, and sustains a vicious cycle of inflammation and disturbed microbiota. The development of holistic analytical approaches to respiratory microbiota may help explain critical phenotypes—like the occurrence of “frequent exacerbators” in chronic lung diseases—and provide novel targets for therapeutic intervention [5].
Despite the multispecies nature of the respiratory microbiota—in which the bacteriome (bacteria), mycobiome (fungi), and virome (viruses) coexist as a larger community—most previous studies have analyzed microbiomes in a singular fashion. To address this research gap, we sought to develop analytical approaches for the integration of microbiomes in order to successfully translate the information that stems from their activity [3]. Accordingly, we sought to build a computational framework to integrate bacterial, fungal, and viral data sets (among others) from well-characterized patient populations in a process that we call “integrative microbiomics” (see Figure 1). Doing so required us to tackle several methodological challenges, such as interindividual variation in the microbiome, compositionality, sparsity, and confounding variables. Our recent review presents an overview of next-generation sequencing approaches for the microbiome—namely targeted and metagenomics sequencing—along with microbiome data analysis techniques [4].
We have successfully applied our integrative microbiomics approach to bronchiectasis exacerbations [1]. Bronchiectasis is a heterogeneous chronic lung disease that causes irreversible dilatation of the airways. The heterogeneity that characterizes this respiratory condition hinders clinical trials and data interpretation. Our efforts were motivated by the following question: Is basing patient stratification on microbiomes a viable strategy? Indeed, bacterial, viral, and fungal communities in bronchiectasis have all been associated with clinical outcomes. However, previous research was based on single-kingdom models wherein microbial overgrowth causes an infection that is then suppressed by antibiotics. Prior bacterial microbiome studies that focused on dominant bacterial taxa revealed minimal actual change during exacerbations (sudden and sustained worsening of a patient’s condition from a stable state). We hypothesized that integrated microbiomes can provide enhanced precision for patient stratification and precision-based therapy given the variability in clinical, immunological, and inflammatory phenotypes, aetiologies, and therapeutic responses.
Figure 1. Overview of the weighted similarity network fusion (WSNF) approach and its application to the integration of microbiomes in a clinical setting. Figure courtesy of the authors.
To test our hypothesis, we proposed a computational approach that integrates microbiomes based on similarity network fusion (SNF) — an idea that originated from genomic-scale data integration [6]. Our framework involves the use of appropriate similarity measures to infer patients’ similarity networks given the properties of the microbiome data sets, including compositionality, high dimensionality, and sparsity. We perform patients’ similarity network inference for each individual microbiome data set (bacteriome, mycobiome, and virome), then employ an SNF approach to combine multiple patient similarity networks into a unified network of patients. To do so, we decompose the similarity network of every microbiome data set into two networks:
- A network that captures the overall network structure, i.e., the similarity of a patient to all other patients
- A network that captures the local network structure, i.e., the similarity of a patient to the \(K\)-most similar patients (where \(K\) is a hyperparameter).
Next, we iteratively fuse the decomposed networks (derived from different microbiome data sets) via a process that is best conceptualized as the diffusion of similarity information through common edges between the different patient similarity networks. To account for the unbalanced nature of the microbiome data sets that arises from the varying quality of reference databases, we use a weighted similarity network fusion (WSNF) approach that assigns weights to microbiomes based on data quality. Our integrative microbiomics pipeline is available as a web tool.
We applied WSNF to evaluate the bronchiectasis microbiome and assessed respiratory specimens that captured bacterial, fungal, and viral taxa from 217 patients. Spectral clustering of the resultant similarity network identified two patient clusters that we mapped onto clinical outcomes, such as frequent exacerbators. Each cluster contained a range of discriminant bacterial, fungal, and viral taxa, thus highlighting the potential for interactions in the observed clinical states [1]. Focusing on the individual cluster, we studied multi-biome microbial interactions by utilizing co-occurrence network analysis; this analysis allowed us to assess interaction networks that involve important respiratory pathogens like Pseudomonas.
More recently, we used our WSNF strategy to investigate potential crosstalk of the gut-lung axis in bronchiectasis [2]. We identified a significant gut-lung interaction within microbial communities during a prospective and concurrent sampling of the gut (stool) and lung (sputum) in 57 patients with bronchiectasis. Multi-biome integration and spectral clustering revealed two patient clusters—characterized by contrasting clinical phenotypes—with different gut-lung interactions. These clinically relevant clusters were not apparent when we examined the lung microbiomes alone. Our analysis hence allowed us to demonstrate that a dysregulated gut-lung axis, driven by lung Pseudomonas, is associated with poorer clinical outcomes in bronchiectasis.
Finally, since microorganisms (bacteria, fungi, viruses, protists, and archaea) live as communities in complex, close environments, we can study their numerous inter- and intra-kingdom interactions with community ecology modeling approaches. Dynamic changes in microbial communities play an important role in human health and disease, and can be significantly altered by the presence of antibiotics. Understanding the governing mechanisms that underlie these dynamics will contribute to the fight against antimicrobial resistance and yield improved treatment plans for patients who suffer from infectious diseases. In the future, we intend to investigate complex microbial interaction patterns in the presence and absence of antibiotics under various environmental conditions. To do so, we will continue to combine mathematical modeling and analysis with wet lab experiments, clinical data, and knowledge.
Krasimira Tsaneva-Atanasova presented this research during an invited presentation at the 2022 SIAM Conference on the Life Sciences, which took place concurrently with the 2022 SIAM Annual Meeting in Pittsburgh, Pa., last year.
References
[1] Aogáin, M.M., Narayana, J.K., Tiew, P.Y, Ali, N.A.B.M, Yong, V.F.L., Jaggi, T.K., … Chotirmall, S.H. (2021). Integrative microbiomics in bronchiectasis exacerbations. Nat. Med., 27, 688-699.
[2] Narayana, J.K., Aliberti, S., Aogáin, M.M., Jaggi, T.K., Ali, N.A.B.M., Ivan, F.X., … Chotirmall, S.H. (2022). Microbial dysregulation of the gut-lung axis in bronchiectasis. Am. J. Respir. Crit. Care Med., 36288294.
[3] Narayana, J.K., Aogáin, M.M., Ali, N.A.B.M., Tsaneva-Atanasova, K., & Chotirmall, S.H. (2021). Similarity network fusion for the integration of multi-omics and microbiomes in respiratory disease. Eur. Respir. J., 58(2), 2101016.
[4] Narayana, J.K., Aogáin, M.M., Goh, W.W.B., Xia, K., Tsaneva-Atanasova, K., & Chotirmall, S.H. (2021). Mathematical-based microbiome analytics for clinical translation. Comput. Struct. Biotechnol. J., 19, 6272-6281.
[5] Narayana, J.K., Tsaneva-Atanasova, K., & Chotirmall, S.H. (2022). Microbiomics-focused data integration: A fresh solve for the Rubik's Cube of endophenotyping? Am. J. Respir. Crit. Care Med., 206(4), 365-368.
[6] Wang, B., Mezlini, A.M., Demir, F., Fiume, M., Tu, Z., Brudno, M., … Goldenberg, A. (2014). Similarity network fusion for aggregating data types on a genomic scale. Nat. Methods, 11, 333-337.
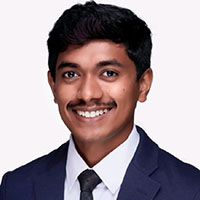 |
Jayanth Kumar Narayana is a doctoral student in mathematical healthcare and precision medicine as part of the joint Nanyang Technological University, Singapore–University of Exeter Ph.D. program, for which he works in the Translational Respiratory Research Lab at LKCMedicine. He received his dual B.S./M.S. degree from the Indian Institute of Science Education and Research, Pune. Narayana’s research focuses on bridging the gap between math and the medical sciences by developing novel data analysis techniques that build on concepts of mathematics and statistical learning to better analyze medical datasets. |
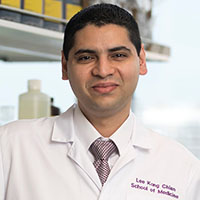 |
Sanjay H. Chotirmall is an internationally recognized clinician-scientist with an established translational respiratory research group at the Lee Kong Chian School of Medicine at Nanyang Technological University, Singapore. He has performed key work on endo-phenotyping pulmonary infection, including the use of next-generation sequencing approaches to study the lung microbiome in the context of chronic inflammatory respiratory diseases. |
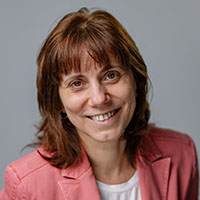 |
Krasimira Tsaneva-Atanasova is a Professor of Mathematics for Healthcare in the Living Systems Institute and the Department of Mathematics and Statistics at the University of Exeter. She received her M.Sc. in mathematics from the University of Plovdiv in Bulgaria and her Ph.D. in applied mathematics from the University of Auckland in New Zealand. Her research and professional activities aim to inform novel applications of mathematics that enable the development of quantitative methods for healthcare and healthcare technologies. |