By Allen Tannenbaum
A deep, quantitative understanding of biomedical systems will aid researchers who are unraveling the underlying mechanisms and pathophysiology of cancer biology. Our goal is to develop detailed models of multiscale tissue morphology and molecular characterization; these models will enable high-precision predictions of tumor change and evolution and help clinicians anticipate treatment responses to combined regimens that consist of radiation therapy, traditional chemotherapy, and targeted therapies. A central issue in this effort is the integration of high-dimensional heterogeneous/hetero-modal data. In order for the datasets to support model development efforts, we must utilize analysis platforms that enable researchers to assemble, query, analyze, and visualize detailed multiscale descriptions of the underlying biological mechanisms in cancer networks. Though the size of datasets and the computational requirements for data analysis and simulation will be extremely large, they must also be compatible with the capabilities of current and planned scale machines. To leverage these systems for cancer modeling, practitioners will need to carry out analysis and visualization many times in situ to explore various initial conditions, extract informative features to develop predictive models, and perform diagnosis and validation of simulations by comparing them to experimental data.
Under the Mathematical Oncology Initiative, our group at Stony Brook University and Memorial Sloan Kettering Cancer Center is applying techniques that are founded upon geometric network analysis to examine some of the aforementioned issues in computational biology, with a particular emphasis on cancer; our ultimate goal is to transfer our work to the clinic. One of the key concepts that we employ is Yann Ollivier’s notion of graph curvature [4], which we refer to as Ollivier-Ricci (OR) curvature. Network geometry—and curvature in particular—is intimately related to network entropy [5, 6]. In fact, one method that extends curvature to rather general metric measure spaces involves exploiting the convexity properties of the entropy along geodesic paths, which one defines at the level of the associated space of probability measures with an induced Riemannian structure [7].
Figure 1. The original optimal mass transport (OMT) problem considered the optimal method for moving a pile of soil or rubble to an excavation. The corresponding metric is known as the Wasserstein distance, Earth Mover’s distance, or simply the optimal mass transport metric. Figure courtesy of the author.
OR curvature itself is based on
optimal mass transport (OMT), a very active and rapidly developing field of research that addresses the geometry of probability densities. Although this is an old research area—initially developed by Gaspard Monge in 1781, with a modern relaxed formulation given by Leonid Kantorovich in 1942—it has undergone a revolution in recent years because of its unique power to handle distributions. OMT has impacted many subjects, such as machine learning, computer vision/image processing, medical imaging analysis, statistical physics, computational biology, econometrics, systems theory, meteorology, transportation, image/signal processing, and expert systems [7].
One can formulate OMT as the problem of transporting one mass to another in a manner that minimizes a given cost functional (see Figure 1). “Mass” is used in a general sense here — in image processing, for example, mass may be represented by intensity. In this case, OMT lends itself to a registration technique; previous work has exploited this direction to find a solution to a partial differential equation [3]. These efforts lead to a family of distances on distributions that generalize and robustify the standard metrics in statistics and data analysis. The metric that is associated with OMT is called the Wasserstein distance (WD), or the Earth Mover’s distance. One very important property of the WD—in contrast to other common metrics on distributions such as Kullback-Leibler divergence, Jensen-Shannon divergence, or total variation—is its (weak) continuity. This feature is especially advantageous since medical data is typically quite noisy.
OMT forms the basis of OR curvature [4]. More precisely, let \((X,d)\) denote a metric space equipped with a family of probability \(\{\mu_x : x \in X\}\). We define the OR curvature \(\kappa(x,y)\) along a geodesic path (shortest distance) that connects nodes \(x\) and \(y\) via \(W_1(\mu_x,\mu_y)=(1-\kappa(x,y))d(x,y)\), where \(W_1\) denotes the Wasserstein \(1\)-distance. For the case of weighted graphs, we set \(d_x=\sum_y w_{xy}\), \(\mu_x(y) := w_{xy}/d_x\), where \(d_x\) is the sum over all neighbors \(y\) of the given code \(x\), and \(w_{xy}\) denotes the weight of an edge that connects node \(x\) with node \(y\). One may regard the measure \(\mu_x\) as the distribution of a one-step random walk that begins at \(x\), where the weight \(w_{xy}\) quantifies the strength of interaction between nodal components or the diffusivity across the corresponding link (edge). Importantly, negative curvature indicates a tree-like structure while positive curvature indicates a more hub-like structure with invariant triangles (or “feedback loops”) at the given node.
Figure 2. Pre-treatment network of the gene expression of 58 cell lines. The network consists of 8,240 nodes (genes) and 67,235 edges. TP53 (with very high absolute curvature) seems to define a key subnetwork within the overall cancer network. Figure courtesy of [5].
The preceding theory’s relationship to cancer biology arises from the fact that one can model many cellular gene and protein networks as weighted graphs whose edges reflect interaction strengths/rates between the corresponding nodes (genes or proteins). As a concrete example, consider a genetic regulatory network. The expression of a gene—i.e., the production of a protein that the given gene encodes—is regulated by other proteins. We can thus model the genomic machine as a graph (network) where vertices represent the genes and edges represent the correlation (dependence) of the corresponding gene’s production of a given protein with the various other proteins that are produced by additional genes in the genome. Figure 2 illustrates this approach for the tumor suppressor gene TP53. TP53 is mutated in more than half of cancers; its very high curvature in a genomic network—which emphasizes its feedback centrality—indicates its importance. This curvature also demonstrates TP53’s functional robustness via the fluctuation theorem from nonequilibrium thermodynamics [2, 6].
Thus, much of our research focuses on the employment of network geometrical concepts to quantify (and therefore predict) pathway-related robustness/fragility in a given cancer system; doing so will uncover hypothesized sets of targets of opportunity that can properly disrupt alternative signaling cascades, which contribute to drug resistance. These analytical methods will hopefully empower treatments that involve targeted drug agents, enable combination of immunotherapy with more traditional chemical agents, and help optimize the efficacy of certain immunotherapy methodologies for the alteration or upregulation of tumor cell antigens. Our approach involves the use of graph theoretic techniques to identify key cancer hubs by partitioning the network into dense, highly connected subgraphs. To account for both the activation and inhibition properties of the various complex interactions, one must extend existing theory to the case of directed graphs [1].
Drug resistance is naturally a major roadblock to successful outcomes in cancer treatment regimens. One key mechanism of resistance stems from the fact that the inhibition of certain critical pathways (i.e., making them more fragile) can cause neighboring pathways to become more robust, thus contributing to an escape route from a given therapy. Network robustness also reflects treatment resistance, and fragility reflects sensitivity. Graph curvature can be quite useful in the quantification of such phenomena, given its strong relationship to network architecture: tree-like (negative) and hub-like (positive). In particular, cancer cells exhibit fate plasticity and are able to shift along a spectrum of differentiation in response to changes in gene expression that are caused by various genetic assaults (radiotherapy, chemotherapy, or immunotherapy) or environmental stresses (hypoxia, reactive oxygen species, etc.). The methods that we propose here can also characterize the processes that lead to differentiation, as well as targeted anticancer therapies that must account for both the differentiation state of the tumor as a whole and the likelihood that drug-resistant sub-clones will emerge.
In summary, the emerging field of mathematical oncology is rapidly becoming an incubator of novel methodologies in the battle against cancer. Hopefully it will have a major impact in helping to drive new treatments for this deadly disease.
Allen Tannenbaum presented this research during a minisymposium at the 2021 SIAM Conference on Optimization, which took place virtually in July in conjunction with the 2021 SIAM Annual Meeting.
References
[1] Alon, U. (2006). An introduction to systems biology: Design principles of biological circuits. Boca Raton, FL: Chapman and Hall/CRC Press.
[2] Demetrius, L., & Manke, T. (2005). Robustness and network evolution — an entropic principle. Phys. A: Stat. Mech. Appl., 346(3-4), 682-696.
[3] Haker, S., Zhu, L., Tannenbaum, A., & Angenent, S. (2004). Optimal mass transport for registration and warping. Int. J. Comput. Vis., 60(3), 225-240.
[4] Ollivier, Y. (2009). Ricci curvature of Markov chains on metric spaces. J. Funct. Anal., 256(3), 810-864.
[5] Pouryahya, M., Oh, J.H., Mathews, J.C., Deasy, J.O., & Tannenbaum, A.R. (2018). Characterizing cancer drug response and biological correlates: A geometric network approach. Sci. Rep., 8, 6402.
[6] Sandhu, R., Georgiou, T., Reznik, E., Zhu, L., Kolesov, I., Senbabaoglu, Y., & Tannenbaum, A. (2015). Graph curvature for differentiating cancer networks. Sci. Rep., 5, 12312.
[7] Villani, C. (2003). Topics in optimal transportation. Providence, RI: American Mathematical Society.
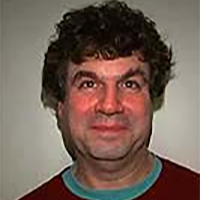
|
Allen Tannenbaum is Distinguished Professor of Computer Science and Applied Mathematics & Statistics at Stony Brook University. He is also an affiliate attending computer scientist in the Department of Medical Physics at Memorial Sloan Kettering Cancer Center.
|