By Lina Sorg
Photosynthesis is the process by which plants absorb solar radiation and carbon dioxide (CO2) from the atmosphere. Some of this radiation gets dissipated as heat during the carbon fixation process, causing the plant to emit red and far-red light. This emission constitutes solar-induced chlorophyll fluorescence (SIF) — an electromagnetic signal emitted by the chlorophyll in plants that measures photosynthetic activity. “The more photosynthetic activity there is, the higher your SIF values are and vice versa,” Manju Johny of NASA Jet Propulsion Laboratory said. Satellites can measure SIF values, which provide insight into crop productivity and changes in vegetation due to climate change and extreme weather events like flood, drought, and fire.
Both extreme weather and general climate change trends can cause disruptions to the plant cycle; such disruptions are evident in changes in photosynthetic activity and carbon cycle dynamics. During a minisymposium presentation at the 2022 SIAM Conference on Mathematics of Data Science, which took place last week in a hybrid format in San Diego, Calif., Johny used data from the NASA’s TROPOspheric Monitoring Instrument (TROPOMI) to monitor SIF values in the Mendocino National Forest and better understand post-wildfire vegetation levels.
The Mendocino National Forest is located in the Coastal Mountain Range in northwestern California and is home to many diverse species of wildlife, some of which are threatened or endangered. Of the forest’s more than 900,000 acres, 60,000 are considered “old growth” — trees that have reached an advanced age without significant disturbance and thus exhibit unique ecological features. “These massive trees can absorb more carbon from the atmosphere and act as a carbon sink,” Johny said. “This is important from the point of view of carbon cycle dynamics.”
In 2018, the Mendocino Complex Fire burned in northern California for nearly three months. It was first reported on July 27, 2018 and was fully contained by September 18th. The wildfire was deemed “complex” because two fires—the Ranch Fire and the River Fire—simultaneously burned in the same general vicinity. The Ranch Fire was California’s single-largest recorded fire at the time and destroyed over 410,000 acres of the forest, devastated the region, and naturally resulted in substantial damage to forest vegetation.
Figure 1. Solar-induced chlorophyll fluorescence (SIF) time series curves that measure photosynthesis in the Mendocino National Forest. The green curve represents the unburned region of the forest and the red curve represents the burned area from the Ranch Fire in 2018. Figure courtesy of Manju Johny.
Given this information, Johny identified two scientific goals for her project: (i) Study the impact of the 2018 Ranch Fire on vegetation in the Mendocino National Forest and (ii) Monitor post-fire vegetation recovery. She began by presenting SIF time series curves that measure photosynthesis in the Mendocino region (see Figure 1). The green curve represents the unburned region of the forest and the red curve represents the burned area from the Ranch Fire. “We are essentially trying to understand whether there is a difference in SIF between the burned and unburned areas,” Johny said. “How can we tell if the observed differences are due to random chance or sampling variability, or whether the two groups are fundamentally distinct?”
One can utilize a statistical hypothesis test to answer this question. The classical one-way ANOVA is inadequate in this scenario, but distinct benefits are associated with the application of a functional data framework. Functional data are curves or surfaces that vary over a continuous domain, can only be collected as discrete measurements in practice, and are almost always observed with error; James Ramsay first coined the term “functional data” in 1982. Johny then introduced functional ANOVA (fANOVA): a resampling-based hypothesis testing framework that compares groups of curves while accounting for dependence.
Researchers use functional data analysis to convert discrete data back into functions through a process called smoothing. There are many ways to smooth data, the two main ones being kernel-based and spline-based approaches. Johny opted for penalized B-spline smoothing and chose the smoothing parameter via generalized cross validation. “For each pixel, we have a function that represents the SIF values over time,” she said.
When applying the fANOVA method, Johny began with two hypotheses. The null hypothesis maintains that the burned and unburned regions of forest have equal population mean functions, while the alternative hypothesis claims that the mean functions for the two group are different. First, Johny obtained the sample mean curve for each group as well as the test statistic (the distance between the sample mean curves), which provides a sense of the difference between the two sample means. She next generated parametric bootstrap resamples under the null hypothesis, repeating the process many times to yield a distribution of Monte Carlo statistics. These statistics represent differences under the null hypothesis. “We calculate our p-value as the proportion of our Monte Carlo statistics that is more extreme than our test statistics,” Johny said. “This gives us an idea of how unusual our observations are when compared to the null hypothesis.”
The generated resample curves must have the same spatio-temporal dependence as the sample data; if not, the type I error (false positive rate) will increase and bring about erroneous conclusions. Because estimating and generating data with complex spatio-temporal dependence is both computationally and theoretically difficult, Johny employed functional principal component analysis to simplify the process. Doing so simplifies the complex two-dimensional estimation and generation problem into two simpler one-dimensional problems; this made it easier for Johny to generate the resample curves, which represent the supposed appearance of the mean difference curves under the null hypothesis. The resulting curves are centered at zero but have the same spatial-temporal dependence as the original sample data.
Figure 2. The functional ANOVA resample curve for the Mendocino National Forest indicated a small p-value and thus a significant decrease in solar-induced chlorophyll fluorescence (SIF) for burned areas when compared to unburned areas. Figure courtesy of Manju Johny.
Johny then provided an illustration of fANOVA to clarify the process. Observed curves that are contained in the middle of the resample curves imply minimal difference between groups and correspond with large p-values. In contrast, observed curves that occur towards the edge of the resample curves are evidence of significant difference between the burned and unburned groups. “This visualization is nice because it tells us things that a single p-value alone can’t,” Johny said.
Her fANOVA-based resample curve indicated a small p-value and thus a significant decrease in SIF for burned areas when compared to unburned areas (see Figure 2). In fact, the SIF in burned regions has still not recovered to pre-fire levels in the Mendocino National Forest. “This shows the utility of using SIF and fANOVA for identifying changes in vegetation and monitoring vegetation recovery,” Johny said. She then repeated the fANOVA test for different parts of the domain, which revealed that the difference in SIF levels in 2019 (one year after the fire) was even greater in magnitude than in 2018 (the same year of the fire). Johny explained that California emerged from a drought in 2019, meaning that unburned areas of the forest flourished while burned areas were just beginning the recovery process. In 2020, a slightly higher p-value indicated that some recovery was underway.
Johny concluded her presentation with a discussion about future steps in the context of SIF data fusion. For example, while she used TROPOMI data in her experiments, she and her colleagues also have access to Orbiting Carbon Observatory-2 (OCO-2) satellite data, which has a finer spatial resolution than TROPOMI but coarser temporal revisits. OCO-2 could provide insight into recovery and vegetation changes over longer time periods as well, since TROPOMI is a newer instrument. “A data fusion between the two could give more reliable SIF estimates for the region,” Johny said.
She also noted that NASA’s 2017 Making Earth System Data Records for Use in Research Environments (MEaSUREs) program provides fused and gap-filled estimates of atmospheric CO2 from multiple instruments. Johny and her colleagues thus plan to add SIF estimates from OCO-2 to the product and utilize it to study the joint behavior of SIF and CO2 during fire events as it relates to the carbon cycle.
Manju Johny of NASA Jet Propulsion Laboratory presented this research during a talk entitled “Spatio-temporal Modeling of Atmospheric Carbon Dioxide and Solar-Induced Chlorophyll Fluorescence” at the 2022 SIAM Conference on Mathematics of Data Science, which took place in San Diego, Calif., from September 26-30, 2022.
Acknowledgments: Other researchers involved in this work include Petrutza Caragea and Kieran Liming of Iowa State University as well as Jonathan Hobbs, Vineet Yadav, Maggie Johnson, Amy Braverman, and Hai Nguyen of the Jet Propulsion Laboratory, which is managed and operated under the California Institute of Technology under contract with NASA. The Making Earth System Data Records for Use in Research Environments (MEaSUREs) program and the Orbiting Carbon Observatory-2 (OCO-2) project also provided support.
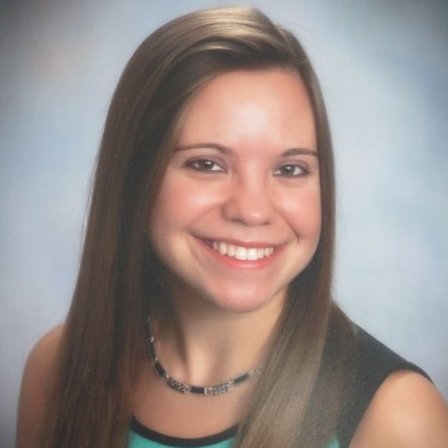 |
Lina Sorg is the managing editor of SIAM News. |