By Anna Ghazaryan and Nancy Rodríguez
The record of human history is awash with civil unrest events in the form of both protests and riots. Their outcomes have varied dramatically — some changed the course of history while others had a negligible impact. The unifying factor of these events is their service as outlets for the expression of objection and dissent towards a legal, political, or social idea, action, or inaction. In the last 15 years, the number of protests around the world has more than tripled [11]. Understanding the mechanisms that drive civil unrest events has become even more important as a result, and mathematical modeling can shed light into these processes.
Researchers have successfully used differential equations to model a variety of social behaviors, such as marital interactions, combat, criminal activity, and opinion formation. In response to growing interest in the analysis of civil unrest, Henri Berestycki, Jean-Pierre Nadal, and Nancy Rodríguez introduced a model that offers insight into the spatiotemporal dynamics of these outbursts [3]. Their model uses existing data—maps, times, the intensity of violence, the number of assaults and burned cars, property damage, and other violent acts—from the 2005 French riots and 2011 London riots, both of which began in response to police brutality [2, 6].
It is now common knowledge that access to social media influences the spread of riots. For instance, the 2011 London riots are sometimes called the BlackBerry riots because BlackBerry smartphones played an instrumental role in the self-organization of rioters. And the events that led to the 2018 Velvet Revolution in Armenia were organized via Facebook. Social media seems to make unrest especially “contagious,” as it spreads both logistical information as well as emotional charge. Naturally, we can model unrest similarly to the spatial spread of a contagious disease.
When investigating the 2005 French riots data, Berestycki and Rodríguez noticed that the peak height of the activity—the highest number of violent events—moved across France [4]. Other studies made the same observation about the 2011 London riots [2, 8]. This phenomenon is mathematically related to the concept of traveling waves, which are observable in real life and can influence nearby dynamics. The mathematical theory of traveling waves finds applications in population dynamics, ecology, combustion theory, biomathematics, and many other areas. A particularly important concept is the stability of waves, which describes their resilience under perturbation.
In their 2015 study, Berestycki and Rodríguez identified two quantities whose evolution they sought to understand: the level of rioting activity or unrest \((u)\) and the measure of effective social tension \((v)\) [4]. Indeed, social dissatisfaction can influence the intensity and duration of social outbursts [9], as was the case during the 2020 George Floyd protests in the U.S. [7]. The level of unrest \(u(x,t)\) measures the amount of activity at any given time \(t\) and spatial location \(x\). One can measure it explicitly — i.e., as the number of people who participate in a protest. The social tension, though not as explicit, is a measure of long-established frustrations due to legal judgements, political decisions, lack of employment opportunities, racial inequality, and gender and other disparities, among other issues. Berestycki, Nadal, and Rodríguez’s collaborative effort to model the interplay between social unrest and social tension resulted in several publications [3-6].
A corresponding model by Berestycki and Rodríguez consists of two coupled reaction-diffusion equations, which are instrumental when examining natural phenomena that result from the interaction of spatial diffusion and the reaction of participating quantities [4]. The system for the level of unrest and social tension is
\[u_t = d_1 u_{xx} + r(v)G(u) − u,\tag1\]
\[v_t = d_2 v_{xx} − \theta h(u)v + 1,\tag2\]
where \(t > 0\) is time and \(x\) is the spatial variable. The terms \(d_1 u_{xx}\) and \(d_2 v_{xx}\) represent the “spread” of the unrest and tension, and the constants \(d_1\) and \(d_2\) are the rates of this spread. Although a two-dimensional spatial variable makes more sense at the modeling level, we can capture many important features by considering a system in one-dimensional space — especially if the analysis is focused on the existence of traveling waves (and particularly planar traveling waves). Traveling waves are special solutions of the underlying equations that preserve their shape while moving in a preferred direction. Here, the traveling waves of interest are those that asymptotically connect two constant states; these types of waves are called fronts. For the system \((1)\)-\((2)\), the constant states are the relaxed state with no activity \((u=0\), \(v=1)\) and the excited state with some activity that is characterized by non-zero values for both variables.
The goal of the function \(G\) is to describe self-excitement or self-reinforcement. Self-reinforcement only occurs when the social tension is sufficiently high; if a social outburst takes place in a community where people are generally satisfied with their lives, rioting behavior is not expected to persist or grow. We therefore introduce a threshold \(a\) as the level of social tension above which self-reinforcement comes into play. The switch for self-reinforcement is modeled as
\[r(v) = \frac{1}{1-e^{\beta(v-a)}}.\tag3\]
Here, the parameter \(\beta > 0\) measures the speed of the transition between the non-excited and excited states.
We assume that the social tension decreases at a rate that is proportional to itself and in a manner that is influenced by the level of rioting behavior. The parameter \(\theta > 0\) calibrates the rioting activity’s influence on the tension. The function \(h\) encodes the effect of the level of activity \(u\) on the tension \(v\):
\[h(u) = (1+u)^p.\]
The parameter \(p\) determines the way in which the level of rioting behavior decreases social tension. The social tension is independent of rioting activity for \(p=0\), meaning that the cases with \(p \ne 0\) are more realistic.
Figure 1. Typical front profiles. 1a. Monotone fronts with a small \(\omega\). 1b. Nonmonotone fronts with a relatively large \(\omega\). Figure courtesy of [1].
When \(p\) is negative, rioting slows the release of tension; as such, rioting activity can enhance social tension. A 2020 study gleaned significant insight into the model dynamics for this tension-enhancing regime, such as the existence and stability of traveling fronts [12]. However, the fronts in this regime are monotone and thus do not present the temporal ups and downs that are evident in actual data for the French riots in 2005.
When \(p\) is positive, social tension decreases more quickly for larger values of \(u\); the rioting behavior releases the built-up social tension and is therefore tension-inhibiting, as was the case with the 2005 French riots. Numerical observations demonstrated that a tension-inhibiting system exhibits both monotone and non-monotone fronts, in agreement with previous data [6, 12]. Yet another study proved the fronts’ existence via the geometric singular perturbation theory in two phenomenologically different situations [1].
In the first of these situations, the rates of the spatial spread of both the level of unrest and social tension are comparably small [1]. The parameter \(\omega\), which sets the timescale over which the bandwagon effect occurs, plays an important role in the proof of the existence of fronts. A realistic interpretation of the constructed solutions is possible. When \(\omega\) is very large, the social tension \(v\) drives the front and the nearby dynamics; when \(\omega\) is very small, the social unrest \(u\) controls the system.
Figure 1 depicts two typical front profiles. Figure 1a illustrates the monotone fronts that manifest for sufficiently small values of \(\omega\), while Figure 1b illustrates the non-monotone fronts that appear for relatively large values of \(\omega\).
Figure 2. Monotone front with sufficiently high speed. Figure courtesy of [1].
The second situation arises when the social tension spreads at a much slower rate than the level of unrest [1]. Traveling fronts exist in this case as well; Figure 2 portrays a monotone front solution that propagates sufficiently fast.
Remarkably, the traveling fronts in system \((1)\)-\((2)\) for this situation are related to the well-understood fronts in Fisher’s equation, which is also known as the Kolmogorov-Petrovsky-Piskunov (KPP) equation. This equation was originally formulated in 1937 to describe propagation of an advantageous gene. It captures spreading phenomena; is often used to model applications in biology, ecology, and sociology; and is fundamental in social applications [1].
Long-established frustrations influence the course of social outbursts [9], and the model that we discuss here inspires a better understanding of this process. Many outbursts of social unrest are initiated by triggering events [10]; therefore, further work could include the analysis of traveling fronts in \((1)\)-\((2)\) in the context of such events. We could even capture externally driven, discontinuous, triggering events by incorporating a sum of weighted Dirac delta functions in \((2)\).
Another possible research direction involves more detailed evaluation of the parameters’ influence on system dynamics. Some rioting or revolutionary events last a few days, while others persist for much longer. Obviously, this kind of human behavior occurs on multiple scales. We must understand the parameters that are responsible for long-term rioting processes—like the 2005 events in Paris and 2011 riots in London—versus shorter outbursts, such as the 2014 unrest in Ferguson, Mo., in response to the shooting of Michael Brown or the 2014 protests in New York related to the chokehold death of Eric Garner. In the future, we may seek to understand whether the shorter duration and the crowd’s failure to self-excite is related to non-existence of a traveling front in the model, or to the instability that causes the front to disappear as a result of a perturbation.
Anna Ghazaryan presented this research during a minisymposium presentation at the 2021 SIAM Conference on Applications of Dynamical Systems, which took place virtually in May 2021.
References
[1] Bakhshi, M., Ghazaryan, A., Manukian, V., & Rodríguez, N. (2021). Traveling wave solutions in a model for social outbursts in a tension-inhibitive regime. Stud. Appl. Math., 147(2), 650-674.
[2] Baudains, P., Braithwaite, A., & Johnson, S.D. (2013). Spatial patterns in the 2011 London riots. Policing, 7(1), 21-31.
[3] Berestycki, H., Nadal, J.-P., & Rodríguez, N. (2015). A model of riot dynamics: Shocks, diffusion and thresholds. Netw. Heterog. Media, 10(3), 443-475.
[4] Berestycki, H., & Rodríguez, N. (2015). Analysis of a heterogeneous model for riot dynamics: The effect of censorship of information. Eur. J. Appl. Math., 27(3), 554-582.
[5] Berestycki, H., Rossi, L., & Rodríguez, N. (2018). Periodic cycles of social outbursts of activity. J. Differ. Equ., 264(1), 163-196.
[6] Bonnasse-Gahot, L., Berestycki, H., Depuiset, M.-A., Gordon, M.B., Roché, S., Rodríguez, N., & Nadal, J.-P. (2018). Epidemiological modelling of the 2005 French riots: A spreading wave and the role of contagion. Sci. Rep., 8, 107.
[7] Cheung, H. (2020, June 8). George Floyd death: Why US protests are so powerful this time. BBC News. Retrieved from https://www.bbc.com/news/world-us-canada-52969905.
[8] Davies, T.P., Fry, H.M., Wilson, A.G., & Bishop, S.R. (2013). A mathematical model of the London riots and their policing. Sci. Rep., 3, 1303.
[9] Lipsky, M. (1968). Protest as a political resource. Am. Polit. Sci. Rev., 62(4), 1144-1158.
[10] Newburn, T. (2014, August 20). The Ferguson riots may seem similar to those in UK in 2011 — but there are stark contrasts. The Guardian. Retrieved from https://www.theguardian.com/commentisfree/2014/aug/20/ferguson-missouri-not-so-far-from-tottenham-toxteth.
[11] Ortiz, I., Burke, S., Berrada, M., & Saenz Cortés, H. (2021). World protests: A study of key protest issues in the 21st century. Cham, Switzerland: Palgrave Macmillan.
[12] Yang, C., & Rodríguez, N. (2020). A numerical perspective on traveling wave solutions in a system for rioting activity. Appl. Math. Comput., 364, 124646.
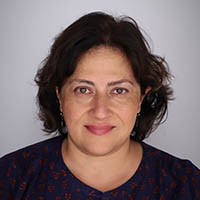 |
Anna Ghazaryan is a professor in the Department of Mathematics at Miami University in Oxford, Ohio. |
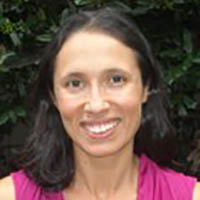 |
Nancy Rodríguez is an associate professor in the Department of Applied Mathematics at the University of Colorado, Boulder. |