By Lina Sorg
Earthquakes occur on the Earth’s surface after a sudden release of energy in the lithosphere generates seismic waves. These phenomena transpire both naturally due to shifting tectonic plates and artificially in response to man-made activity, such as gas extraction (as is common in the Netherlands). But regardless of their origin, earthquakes cause considerable damage worldwide. According to the Federal Emergency Management Agency, earthquake-related losses in the U.S. amount to roughly $4.4 billion each year. And the 2011 Tōhoku earthquake, which hit Japan in 2011 and triggered a tsunami, caused more than $100 billion worth of damage. Given the severity of the financial, social, and economic consequences of earthquakes, better forecasting methods are both desirable and necessary.
Yet forecasting earthquakes is quite challenging because the seismic cycle is irregular, difficult to predict, and comprised of multiple different phases (see Figure 1). For instance, preexisting faults in the Earth’s subsurface experience high levels of shear stress and begin to slip during the nucleation phase. Earthquakes take place in the coseismic phase, when the built-up levels of shear stress suddenly and rapidly drop. The faults begin to recover in the postseismic and interseismic phases, after which stress continues to reaccumulate until the next event is eventually triggered.
Figure 1. Explanation of the various phases of the seismic cycle. Figure courtesy of Hamed Diab-Montero.
During a minisymposium presentation at the 2022 SIAM Conference on Mathematics of Data Science, which was held in a hybrid format in San Diego, Calif., last week, Femke Vossepoel of Delft University of Technology utilized data assimilation techniques, including an ensemble Kalman filter (EnKF), to simulate earthquake recurrence and improve forecasting methods. She focused on the work of Hamed Diab-Montero, a Ph.D. student at Delft who paired the aforementioned approaches with a seismo-thermo-mechanical (STM) model. Vossepoel and Diab-Montero collaborate in this project with seismologists Ylona van Dinther and André Niemeijer of Utrecht University (also in the Netherlands).
STM models are computationally expensive and account for conservation of mass and momentum, pressure, and temperature, among other variables. When a fault slips, the fault’s stress depends on friction, which in turn depends on the so-called state of the fault. Vossepoel clarified that the “state” in seismology is not a state vector; instead, it is a scalar that indicates increases during the interseismic phase (stick) and decreases during the coseismic phase (slip). Other variables of importance in the model include the slip (deformation along the fault), slip rate, and shear stress at the fault.
However, models alone cannot effectively forecast earthquakes. “We need information to feed our models,” Vossepoel said. This information comes from various sources that measure surface deformation and vibration, as well as measurements of the location of faults and layers. Possible sources include satellite-based images, seismic data, subsurface sensor observations, and even cables on the ocean floor. “Most of these observations are indirect,” Vossepoel said. “In order to really know what’s going on, you ideally want to have measurements at the fault.” Because researchers typically do not have access to such measurements, they use laboratory setups to sense the fault activity.
Figure 2. Description of the logistics of the identical twin experiment. Figure courtesy of Hamed Diab-Montero.
Next, Vossepoel discussed an elaborate laboratory setup by a group in Japan with whom the project team is collaborating. “It’s able to simulate earthquakes quite well,” Vossepoel said. “It’s not exactly at field scale, but it’s at a larger scale than previous lab experiments. It’s attractive to use data from a lab because we can actually see and measure what’s happening at the fault interface.” The setup—which involves an upper and lower sandstone block—allows the researchers to sense the stresses at points that are close to the seismogenic zone, though not at the zone itself.
The STM model employed an EnKF with 50 ensemble members to estimate shear stress at the fault, slip velocity, and the state variable. To do so, Diab-Montero relied on a simulator called GARNET: an open-source, multimodal code. “Initially the experiments were simulated on slow-slip events, but now we’re also simulating data into earthquake events,” Vossepoel said. “The ultimate goal is to mimic the lab experiments in Japan.” Forecasting slow-slip events is much easier than forecasting earthquakes because slip events—failures of the fault—occur much more gradually in slow-slip situations.
Vossepoel shared results that were based on identical twin experiments (see Figure 2). “In the lack of real observations, we actually generated a synthetic truth, then we generated synthetic observations by adding noise to the truth,” she said. “So we generated shear stress and velocity and assimilated that into our models, where we have a prior distribution of shear stress at the fault given by our assumptions of the uncertainty of the shear stress.”
Figure 3. Molchan diagram that tracks alarm duration and failure to predict when 10, 20, and 30 percent of ensemble members exceed a certain threshold value of shear stress. Data assimilation techniques perform much better than random forecast. Figure courtesy of Hamed Diab-Montero.
Diab-Montero used the synthetic earthquake tests to create a Molchan diagram to track seismic forecast information (see Figure 3). The horizonal axis of these diagrams measures the alarm duration; this value would be 1 if an earthquake warning was issued every day. Prediction failure falls along the vertical axis. One naturally wants both alarm duration and prediction failure to be low, so that alarms are not unnecessarily startling residents and predictions are as accurate as possible. Diab-Montero’s diagram specifically tracks the potential outcome of 10, 20, and 30 percent of ensemble members exceeding a certain threshold value of shear stress, which would sound an alarm for each of those points. “In all of these cases, you see that the data assimilation has really improved compared to a random forecast,” Vossepoel said. The same is true when assimilation is compared to a periodic model.
Ultimately, the outcome of this work demonstrates that data assimilation effectively improves the simulation and forecast of earthquakes in STM models. The team is currently attempting to move the synthetic twin experiments to actual experiments with laboratory data, as well as explore the possible use of the particle flow filter data assimilation technique. “The use of data assimilation in earthquake modeling is still very much in its infancy, and there’s still a lot of discover,” Vossepoel said. “That’s what makes it for me a very exciting domain of research.”
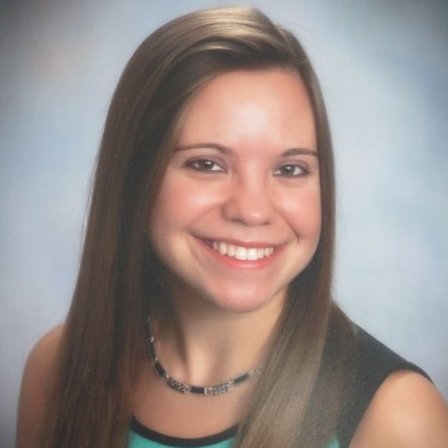
|
Lina Sorg is the managing editor of SIAM News. |