By Lina Sorg
Accurate models can further researchers’ overall knowledge of cerebrovascular diseases like stroke and intracranial aneurysm, which arise due to issues with blood vessels in the brain (see Figure 1). The Circle of Willis (CoW), which is located at the brain’s base, is the main vessel structure that supplies blood to many regions of the brain and its surrounding structures. “A blood clot in this vessel structure can impair brain function and cognitive performance, potentially leading to ischemic stroke,” Sahani Pathiraja of the University of New South Wales said. The CoW’s anatomy—in terms of the width of key arteries in the vessel structure or the density of the connecting vessels—is highly variable between patients. Modelers must therefore rely on patient-specific modeling to better understand cerebrovascular disease.
Figure 1. Examples of cerebrovascular diseases that arise due to issues with blood vessels in the brain. Figure courtesy of Sahani Pathiraja.
Hemodynamic models examine the blood flow of specific vessel structures in the brain. They typically yield a time series of flow or velocity fields that scientists can then use to compute other variables of interest, such as pressure and shear stress (which is responsible for weakening vessel walls and aneurysm formation). One important data source for this type of modeling is magnetic resonance imaging (MRI). For example, researchers use phase contrast (PC)-MRI to obtain measurements of blood flow fields in three dimensions. However, PC-MRI data usually have a low signal-to-noise ratio and poor spatial and temporal resolution due to the time it takes to acquire the necessary measurements.
Because most steps in the hemodynamic modeling process incur some type of error, one cannot rely on these approaches alone for the estimation of flow fields. The aforementioned limitations make this problem a prime candidate for data assimilation, which involves optimally combining uncertain data and models. During a minisymposium presentation at the 2022 SIAM Conference on Mathematics of Data Science, which is currently taking place in a hybrid format in San Diego, Calif., Pathiraja discussed work—undertaken together with her co-authors Franziska Gaidzik, Soumick Chatterjee, et al. from the Otto Von Guericke University Magdeburg—on the utility of sequential data assimilation methods for hemodynamic modeling. She also sought to efficiently and accurately generate samples of image segmentations to capture uncertainty in the model geometry.
Pathiraja and her colleagues focused on the major arteries of the CoW, as segmentation of the minor vessels is quite challenging (see Figure 2). Obtaining the width and spatial arrangement of the arteries is crucial in order to accurately model activity in a patient’s brain. They utilized classical incompressible three-dimensional (3D) Navier-Stokes equations with constant viscosity and standard no-slip boundary conditions. For the corresponding simulation, Pathiraja and her co-authors opted for the 3D localized ensemble transform Kalman filter (LETKF) with 25 ensemble members. “Accurate uncertainty quantification (UQ) in both the model and the observations is critical because this is what ultimately determines what source of information is given higher weight by the ensemble Kalman method,” Pathiraja said, nothing that the use of UQ has room for improvement based on certain assumptions.
She then shared a small subset of results from the data assimilation in the form of two-dimensional velocity profiles and a contour plot of wall shear stress, both at an instantaneous point in time. Though there is no ground truth for this experiment, the data’s varying impact on the assimilation is evident. “It appears to have a stronger weight in the velocity profile of the middle cerebral artery compared to the anterior artery, where the model has stronger influence,” Pathiraja said. In the context of wall shear stress, which helps determine the formation of aneurysms, variations emerge in the location of very high shear stress. “The point is that the data assimilation has the potential to provide improved estimates, so long as the UQ is performed appropriately,” Pathiraja continued. She also shared recent work by her and colleagues that proposed an improved variational autoencoder-based method for UQ of neural network (NN)-based image segmentation methods.
Figure 2. The major arteries within the Circle of Willis, which is the main vessel structure that supplies blood to the brain. Figure courtesy of Sahani Pathiraja.
In the second part of her talk, Pathiraja explored probabilistic machine learning (ML) methods for automatic segmentation of MRI images. “Segmentation in this context refers to two-dimensional (2D) or 3D images and classifying each of the pixels or voxels as either a vessel or not,” she said. “So it’s essentially a binary classification problem at the pixel level.” Traditional segmentation methods frequently involve either (i) pure manual segmentation by a medical expert with prior knowledge of typical vessel structures, or (ii) semi-automatic methods like the Frangi filter, which enhances an image to emphasize the vessel-like structures. Both forms are quite tedious or require some sort of tuning.
Previous years have seen a surge in ML for the segmentation of medical images. Typically, such techniques are NN-type methods that require thousands of images for training purposes, which is largely infeasible in a medical setting. As such, researchers developed specific NN architectures for the small data regime. One such approach is the U-Net: a U-shaped convolutional NN (CNN) for biomedical image segmentation. “The weights of all the convolution kernels are trained with something like stochastic gradient descent, with an appropriate loss function,” Pathiraja said.
Given the U-Net’s popularity, scientists proposed an extension to allow for the generation of samples of image segmentations. This method combines a conditional variational autoencoder with a U-Net; the variational autoencoder learns the statistical properties of a lower-dimensional representation of the segmentation. “The actual segmentation is still performed by the U-Net itself, but it’s influenced by a sampled latent vector that is incorporated into the second-to-last layer of the U-Net,” Pathiraja said.
A crucial feature of the probabilistic U-Net is that it makes use of cross entropy as a loss function, which can render the U-Net unstable for training purposes. Pathiraja therefore considered several alternative loss functions that better utilize distributional information. To test these variations, she designed a synthetic segmentation process that uses a Frangi filter and allows her to verify the methods against a true distribution. Pathiraja relied on a data set of nearly 2,000 2D slices of time of flight MRI brain scans and—using her synthetic segmentation method—generated 10 plausible segmentations per image and evaluated the resulting formulations on a test data set. The methods based on the proposed improvements perform significantly better than the original probabilistic U-Net, though they do take much longer to train.
Pathiraja concluded her presentation with a brief discussion of next steps for this research. For instance, she and her colleagues are working to determine whether their results can extend to a 3D setting as well. “There is still more to be done in improving efficiency and exploring different architectures,” she said. “We’re also planning to verify these methods on a real data set consisting of more realistic plausible segmentations for each image.”
Acknowledgments: This work was undertaken with Franziska Gaidzik, Soumick Chatterjee, Sylvia Saalfeld, Daniel Stucht, Oliver Speck (also Leibniz Institute for Neurobiology Germany), Dominique Thevenin, Gabor Janiga, and Andreas Nuernberger (all from Otto Von Guericke University Magdeburg). Funding from the Deutsche Forschungsgemeinschaft (DFG) Collaborative Research Centre on Data Assimilation (Project -ID 318763901 - SFB1294) and European Structural Investment Funds (project no. ZS/2016/08/80646)—as well as the Forschungscampus STIMULATE (I-117) and DFG Grant (SA 3461/2-1)—is gratefully acknowledged.
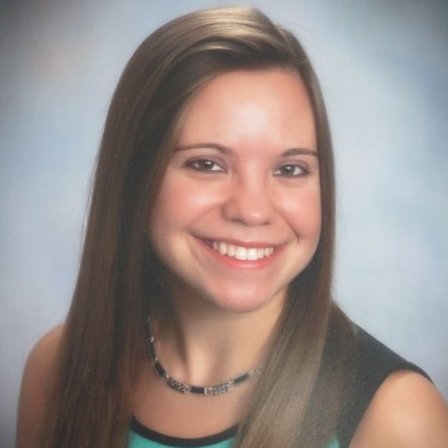 |
Lina Sorg is the managing editor of SIAM News. |