By Behdad Chalaki and Andreas A. Malikopoulos
Traffic congestion is a significant concern in large urban areas around the world. In 2017, congestion in urban areas across the U.S. led to drivers collectively spending an extra 8.8 billion hours on the road and purchasing an additional 3.3 billion gallons of fuel, ultimately resulting in a $166 billion expense [10]. According to the National Highway Traffic Safety Administration, motor vehicle crashes in 2010 cost the U.S. $594 billion due to loss of life and decreased quality of life as a result of injuries.
Connected and automated vehicles (CAVs) are expected to improve both traffic throughput and passenger safety due to their on-board computing capabilities and advanced communication technologies. If CAVs coordinate with each other in traffic scenarios—e.g., by crossing signal-free intersections—they can smooth traffic flow and eliminate stop-and-go driving within the transportation network [6, 8, 13]. Two recent survey papers provide thorough discussions of the research efforts that pertain to the control and coordination of CAVs in the current literature [4, 9].
Although several studies have established a coordination framework for CAVs at single signal-free intersections, a limited number of papers have reported results on multiple adjacent intersections. One common approach to ensure safety involves the use of a first-in-first-out (FIFO) queuing policy to find the crossing order for CAVs that enter the area of potential lateral collision, which is called the merging zone [1, 7, 11, 12]. However, employment of the same policy for adjacent intersections may yield suboptimal solutions and unnecessary CAV slowdowns on the roads that connect the intersections.
Figure 1. Three interconnected intersections that are partitioned into different zones. Figure courtesy of Behdad Chalaki.
In this article, we briefly review our bi-level hierarchical decentralized coordination framework for CAVs at adjacent intersections with the goal of minimizing total travel time (see Figure 1). When they are inside of a control zone, the CAVs can communicate with a coordinator. The coordinator serves as a database among the CAVs and is not involved in any decision-making. Upon entering the control zone, each CAV communicates with the coordinator to access the planned trajectories of CAVs that are already in the control zone. After obtaining this information, the CAV derives its time-optimal trajectory via our bi-level hierarchical coordination framework. This trajectory is then conveyed back to the coordinator so that subsequent CAVs receive the necessary information and can plan their trajectories accordingly.
Our hierarchical coordination framework [3] for CAVs consists of an upper-level problem and a low-level optimal control problem. We partition the roads around the intersections into several zones and assume that each CAV’s speed is given at the zone boundaries that fall inside the merging zones. We formulate a scheduling problem for every CAV within the upper-level problem; the solution of this scheduling problem computes the CAV’s arrival time to each zone on its path in a manner that guarantees safety constraints while minimizing the total travel time inside the control zone. Once they enter the control zone, every CAV first solves two sub-problems: a release-time problem and a deadline problem. The solutions of these two problems respectively determine the earliest and latest feasible arrival times at each zone. To improve computational efficiency in the upper-level problem, we apply a Hamiltonian analysis [2] that yields the earliest and latest feasible arrival times for CAVs by only considering the bounds on their speed and control inputs (acceleration/deceleration) without accounting for any safety constraints.
Figure 2. The hierarchical system architecture for connected and automated vehicle (CAV) \(i\) and the coordinator. Figure courtesy of Behdad Chalaki.
Each CAV uses these solutions to convert its scheduling problem—which explicitly considers all safety constraints—to a mixed-integer linear programming problem. We can solve the latter with standard commercial solvers like CPLEX [5]. The solution of the upper-level scheduling problem becomes the input of the low-level problem for each CAV. The low-level problem then yields the minimum control input at each zone (acceleration/deceleration) that satisfies both the time that is specified in the upper-level scheduling problem as well as the speed-dependent rear-end safety and state/control constraints. Figure 2 illustrates this bi-level hierarchical architecture.
Animation 1 demonstrates our coordination framework for 120 CAVs that are entering two adjacent intersections from 30 different paths based on the Poisson distribution with a rate of one second. A complete analysis of our framework and our simulation results on comparison with signalized intersections, centralized scheduling, and FIFO queuing policy is available in our corresponding paper [3].
Animation 1. Coordination of connected and automated vehicles (CAVs) at two adjacent intersections. Courtesy of Behdad Chalaki [3].
Behdad Chalaki presented this research during a contributed presentation at the 2021 SIAM Conference on Control and Its Applications, which took place virtually in July in conjunction with the 2021 SIAM Annual Meeting.
Acknowledgments: This research was supported in part by the Delaware Energy Institute and ARPA-E’s NEXTCAR program under award number DE-AR0000796.
References
[1] Bichiou, Y., & Rakha, H.A. (2018). Developing an optimal intersection control system for automated connected vehicles. IEEE Trans. Intell. Transport. Syst., 20, 1908-1916.
[2] Bryson, A.E., & Ho, Y.C. (1975). Applied optimal control: Optimization, estimation and control. New York, NY: Taylor & Francis Group.
[3] Chalaki, B., & Malikopoulos, A.A. (2021). Time-optimal coordination for connected and automated vehicles at adjacent intersections. IEEE Trans. Intell. Transport. Syst. To appear.
[4] Guanetti, J., Kim, Y., & Borrelli, F. (2018). Control of connected and automated vehicles: State of the art and future challenges. Ann. Rev. Control, 45, 18-40.
[5] International Business Machines Corporation. (2019). IBM ILOG CPLEX Optimization Studio V12.10.0 documentation. Armonk, NY: IBM.
[6] Klein, I., & Ben-Elia, E. (2016). Emergence of cooperation in congested road networks using ICT and future and emerging technologies: A game-based review. Transport. Res. Part C: Emerg. Tech., 72, 10-28.
[7] Malikopoulos, A.A., Cassandras, C.G., & Zhang, Y. (2018). A decentralized energy-optimal control framework for connected automated vehicles at signal-free intersections. Automatica, 93, 244-256.
[8] Melo, S., Macedo, J., & Baptista, P. (2017). Guiding cities to pursue a smart mobility paradigm: An example from vehicle routing guidance and its traffic and operational effects. Res. Transport. Econ., 65, 24-33.
[9] Rios-Torres, J., & Malikopoulos, A.A. (2016). A survey on the coordination of connected and automated vehicles at intersections and merging at highway on-ramps. IEEE Trans. Intell. Transport. Syst., 18, 1066-1077.
[10] Schrank, B., Eisele, B., & Lomax, T. (2019). Urban mobility report 2019. Texas A&M Transportation Institute.
[11] Zhang, Y., & Cassandras, C.G. (2019). Decentralized optimal control of connected automated vehicles at signal-free intersections including comfort-constrained turns and safety guarantees. Automatica, 109, 108563.
[12] Zhang, Y., & Cassandras, C.G. (2019). Joint time and energy-optimal control of connected automated vehicles at signal-free intersections with speed-dependent safety guarantees. In 2019 IEEE 58th conference on decision and control (CDC) (pp. 329-334). Nice, France: Institute of Electrical and Electronics Engineers.
[13] Zhao, L., & Malikopoulos, A.A. (2021). Enhanced mobility with connectivity and automation: A review of shared autonomous vehicle systems. IEEE Intell. Transport. Syst. Mag. In press.
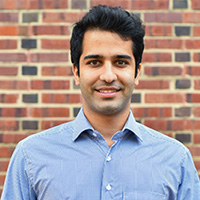
|
Behdad Chalaki is currently a Ph.D. candidate in the Department of Mechanical Engineering at the University of Delaware. His primary research interests lie at the intersections of decentralized optimal control, statistics, and machine learning, with an emphasis on transportation networks. Chalaki is particularly motivated by problems that are related to the improvement of traffic efficiency and safety in smart cities via optimization techniques.
|
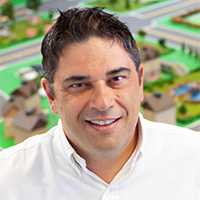
|
Andreas A. Malikopoulos is the Terri Connor Kelly and John Kelly Career Development Associate Professor in the Department of Mechanical Engineering at the University of Delaware. His research spans several fields, including analysis, optimization, and control of cyber-physical systems; decentralized systems; stochastic scheduling and resource allocation problems; and learning in complex systems. The emphasis is on applications that are related to smart cities, emerging mobility systems, and sociotechnical systems.
|