By Anna Seigal
The new SIAM Journal on Applied Algebra and Geometry (SIAGA).
SIAM’s brand-new journal, the SIAM Journal on Applied Algebra and Geometry, will feature exceptional research on the development of algebraic, geometric, and topological methods with strong connections to applications. The cover of the new journal shows seven pictures. By describing these pictures and discussing the topics they represent, we hope to give readers a glimpse into the world of algebraic, geometric, and topological problems of interest to applied mathematicians.
This article is part one of a three-part series. View parts two and three.
1. Polynomial Optimization
The Context
Optimization is a central pillar of applied mathematics, with applications in fields ranging from biology to engineering to finance. Polynomial optimization involves maximizing or minimizing a polynomial function subject to constraints given by polynomial equations. The feasible region, or the set of points in space that satisfy the required constraints, is our geometric object of interest. When considering particular structure on the constraints of an optimization problem, we have corresponding information on the kind of shape the feasible region can take. The boundary of the feasible region is of particular importance, since this is where the optimizing solution will often be found.
The geometry of a feasible region elucidates important aspects of the optimization problem. For example, it may indicate the type of algorithm best suited to maximize a function on that shape. In return, the family of geometrical shapes naturally associated with an optimization problem connects it to the expertise of other areas of mathematics.
Figure 1. Finding the solution to a polynomial optimization problem.
Semi-definite optimization is a generalization of linear optimization. Here, we extend from constraints given by positivity conditions on the entries of a vector to the notion of positivity for a matrix. The constraints take the form of positive semi-definiteness of real symmetric matrices. Feasible regions take the form of spectrahedra, which result from intersecting the space of positive definite matrices with a linear space. Semi-definite optimization problems are often valuable stepping-stones in understanding more complicated problems, and can be used as relaxations of the harder problems. For more on semi-definite optimization and its connection to algebraic geometry, see [2].
The Figure
A naturally-occurring feasible region with a nonlinear boundary is the circle \[{(x-u_1)}^2+ {(y-v_1)}^2= d^2,\] the collection of points a fixed distance away from the center.
Perhaps instead we want to be a fixed distance away from two points – close to both the train station and the ferry terminal, for example. The collection of points whose sum of distances from two points is a constant describes an ellipse \[\bigg\{t (x,y) \in \mathbb{R}^2 : \overset{2}{\underset{i=1}{\Sigma}} \sqrt{(x-u_i)^2 + (y-v_i)^2} = d.\bigg\} \] For problems involving, say, three factories, five train stations, and a ferry terminal, we want to generalize this notion to the so-called \(k\)-ellipse. This is the set of points whose sum of distances from k given points is equal to some constant.
We fix the locations of \(k\) focal points \(u_i, v_i\) and consider the distance \(d\) to be an unknown. Figure 1 shows the surface \[\bigg \{ (x,y,d) \in \mathbb{R}^3 : \overset{k}{\underset{i=1}{\Sigma}} \sqrt{(x-u_i)^2 + (y-v_i)^2} = d \bigg \}\] of points for which the sum of the distances from \((x, y)\) to the focal points is \(d\). A polynomial equation can describe this constraint, which is the nonlinear boundary of the feasible region in a particular semi-definite optimization problem.
The central convex part of Figure 1 holds the solution to this minimization problem. Its lowest point is the Fermat-Weber point, which is often sought. If the distance of interest, \(d\), is fixed, then we take a horizontal slice through the picture and optimize on this slice. The external components are also algebraic solutions to the polynomial constraints.
Figure 1 first appeared in [4], and this version of the image is due to Cynthia Vinzant. It appeared as cover art for the May 2014 issue of the American Mathematical Society’s Notices.
2. Robotics
The Context
Figure 2. Polynomial movement in robotics.
Robotics—the design of mechanical machines to perform complex tasks—is a burgeoning field of study. The range and precision of robots’ motion are limited by the mechanics of their constituent parts, which traditionally consist of rigid pieces connected by joints. The interplay between robotics, algebra, and geometry arises naturally from the kinematics of these pieces working together. In fact, numerical algebraic geometry largely arose from these applications. For an introduction, see Chapter 6 of [3].
A rigid part floating unconstrained in three-dimensional space has six degrees of freedom, but the joints of a mechanism restrict its motion. For most joints used in robotics, polynomial equations can describe these restrictions. With multiple pieces working together, one task is to find the location of a selected terminating part. For example, for fixed locations and angles of your shoulder, elbow, wrist, and carpometacarpal joint, what is the location of your thumb? This is known as forward kinematics. Furthermore, given a desired location of your thumb, what are the possible angles of your arm and hand that would achieve that location? This is inverse kinematics.
The numerical algebraic geometry tool of homotopy continuation can help answer these questions. In homotopy continuation, we first solve an easier but related set of polynomial equations. Then we deform the easier system to the more challenging problem of interest via a homotopy map. We use the solutions of the easier problem to obtain those of the harder problem by numerically tracking the paths of the original solutions as they deform under the homotopy. For more, see [1].
The Figure
Figure 2 depicts a special combination of rigid pieces and joints called a Griffis-Duffy Type I Platform. It consists of two equilateral triangles, one fixed at the base and the other held above it by six rigid legs. Each leg connects a vertex of one triangle to a midpoint of an edge on the other. Although the lengths of each leg are fixed, the angles at each joint are free to move.
The geometry of this problem yields a system of polynomial equations that describes its kinematics: if we fix the point shown on top of the upper triangle, the collection of positions it can reach is the red curve, which is an algebraic set of degree 40.
Figure 2 was created by Charles Wampler of General Motors and Douglas Arnold of the University of Minnesota. It appeared on the poster for the IMA Thematic Year on Applications of Algebraic Geometry in 2006-07, in which significant progress was made connecting the use of algebraic geometry tools to industrial and applied mathematics.
References
[1] Bates, D.J., Hauenstein, J.D., Sommese, A.J., & Wampler, C.W. (2013). Numerically Solving Polynomial Systems with Bertini. Philadelphia, PA: Society for Industrial and Applied Mathematics.
[2] Blekherman, G., Parrilo, P., & Thomas, R. (2013). Semidefinite Optimization and Convex Algebraic Geometry. MOS-SIAM Series on Optimization. Philadelphia, PA: Society for Industrial and Applied Mathematics.
[3] Cox, D., Little, J., & O’Shea, D. (2007). Ideals, Varieties and Algorithms: An Introduction to Computational Algebraic Geometry and Commutative Algebra. New York, NY: Springer Science+Business Media.
[4] Nie, J., Parrilo, P., & Sturmfels, B. (2008). Semidefinite representation of the k-ellipse. In Algorithms in Algebraic Geometry (pp. 117-132). The IMA Volumes in Mathematics and its Applications, book 146. New York, NY: Springer Science+Business Media.
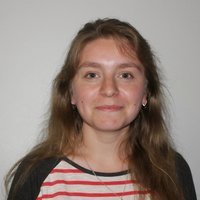 |
Anna Seigal is a graduate student at the University of California, Berkeley, working in applied algebra. Her interests lie in tensors and applications to biological data. She enjoys writing about mathematics in terms of pictures, and blogs on this subject at https://picturethismaths.wordpress.com/. |