Quantifying Social and Nonsocial Influences in Substance Use Disorder
By Lina Sorg
The current U.S. opioid epidemic began in the late 1990s as a result of lobbyist groups’ complaints that doctors were consistently undertreating pain, combined with claims from pharmaceutical companies that drugs like oxycontin were less addictive than other prescription painkillers. In response, doctors began to prescribe opioids at unprecedented rates and the painkillers were abundantly available in the 2000s. The growing dependence on—and consequent abuse of—opioids eventually led to heroin abuse; for instance, more than 80 percent of heroin users in a 2008 to 2010 study reported nonmedical use of prescription opioid drugs in the year before they turned to heroin. 2018 and 2019 saw a second shift to an illicitly manufactured fentanyl-based market. Fentanyl is 50 to 100 times more potent than morphine, and dealers increased their own profits but cutting their products with fentanyl. Figure 1 depicts the three waves of opioid and heroin overdose deaths beginning in 1999. As evidenced by the graph, deaths from synthetic opioid overdose (specifically fentanyl) are still very much on the rise.
During a minisymposium presentation at the 2023 SIAM Conference on Control and Its Applications, which is currently taking place in Philadelphia, Pa., Leigh Pearcy of the University of Tennessee utilized optimal control theory to account for both social and nonsocial paths to addiction and determine effective management strategies for substance use disorder (SUD). She began by introducing a 2019 opioid model with four classes: susceptible (S), prescribed (P), addicted (A), and rehabilitation (R). This model relies upon a standard susceptible-infected-recovered (SIR) framework and comprises a system of ordinary differential equations. Each variable is modeled as a proportion of the total population. Pearcy operates under the following model assumptions:
- The birth rate is equal to the total death rate
- The prescription rate and completion rates are linear
- Both social and nonsocial routes can lead to the development of SUD.
Figure 1. Three waves of opioid overdose deaths. Figure courtesy of the National Vital Statistics System Mortality File.
Next, she explained the difference between social and nonsocial routes to addiction. Social routes mimic the standard progression in a typical disease model, in that addictive behavior spreads through social interactions. But some people develop SUD without social influence—for example, through trauma exposure, mental illness, genetic predisposition, or other external factors—and rely on stolen prescription pills from friends or family in nonsocial settings.
Researchers often model addiction with an infectious disease framework and assume that addictive behaviors spread only through direct social contact. However, this framework does not account for those who develop addiction through nonsocial means. “We’re interested in understanding how nonsocial development of SUD affects model dynamics,” Pearcy said.
So, she and her collaborators went back to the basics and started with adapted versions of the classic susceptible-infectious-susceptible (SIS) and SIR disease models (see Figure 2). They extended both models by adding a spontaneous non-contact linear addiction rate (i.e., a nonsocial addiction pathway), which fundamentally changes the model dynamics and accounts for the nonsocial spread of addiction. The team sought to eliminate the proportion of SUD in a population by reducing the addiction rates \(\beta\) (social rate of development) and \(\epsilon\) (nonsocial rate of development). “If we’re trying to minimize addiction in a population, should we try to reduce \(\beta\) or \(\epsilon\)?” Pearcy asked.
Figure 2. Compartmental diagrams for the susceptible-use disorder-susceptible (SUS) and susceptible-use disorder-recovered (SUR) models of substance use disorder. Figure courtesy of Leigh Pearcy.
This question invokes optimal control theory. Pearcy assumes that a fixed amount of resources (monetary or otherwise) \(\kappa\) is allotted for the reduction of SUD. She then had to decide whether to put these resources towards \(\beta\) or \(\epsilon\). “We can kind of play with the proportional response constants depending on the known factors,” she said.
Pearcy and her colleagues determined that if the overall level of SUD is sufficiently small, the model should focus on reducing the nonsocial rate \(\epsilon\). But when the state level crosses a certain threshold, efforts should instead concentrate on reducing the social rate \(\beta\). This threshold exists in both the susceptible-use disorder-susceptible (SUS) and susceptible-use disorder-recovered (SUR) models. They tested their optimal control solution against other control strategies—including the reduction of only \(\beta\) or \(\epsilon\) and the equal division of efforts between the two—and found that it performed better than these existing methods.
To extend this work, Pearcy plans to examine the effect of linear or nonsocial development of SUD in other disease models, such as susceptible-exposed-infected-recovered (SEIR); explore other types of control constraints for added flexibility; and generalize the control formulation to other SUD models, including the 2019 SPAR opioid model.
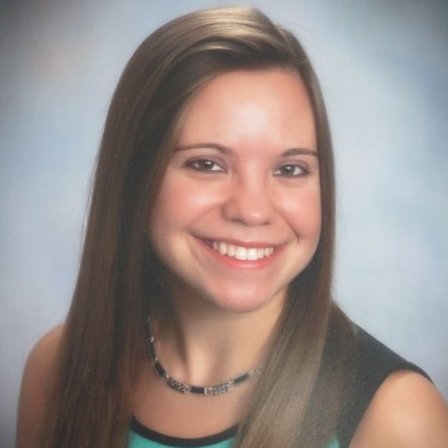
|
Lina Sorg is the managing editor of SIAM News. |