Glacial Lake Outburst Floods and Lava Domes Provide Context for Coupled Physical Models
By Lina Sorg
Mathematical models often involve multiple physical processes that operate at different length and time scales and require prolonged simulation. Researchers can couple these processes within physical models in several ways. During a minisymposium talk at the 2021 SIAM Conference on Computational Science and Engineering, which took place virtually this week, E. Bruce Pitman of the State University of New York at Buffalo offered a general overview of emulators in coupled physical models.
First, consider a glacial lake outburst flood (GLOF) that results from the failure of a moraine-dammed lake. GLOF initiates a cascade of events. When a glacial lake’s natural moraine bounding (consisting of glacial sediment) fails, the resulting floodwater gushes downstream in the form of an aggressive shallow water flow. Moraine failure can release up to 106 cubic meters of water, which bulks as it goes. The rapid surge of water, mud, and other debris may reach speeds of up to 80 meters per second and pose a significant hazard for inhabitants of the nearby mountain communities.
On October 7, 1994, a moraine failure at Lugge Lake in the Luana region of Bhutan caused a GLOF. The glacial floodwater poured into an existing older lake and overwhelmed a small village, killing more than 20 people. A similar situation occurred just last month when the snout of a glacier in the Himalayas broke off and released glacial water and ice. The surge flowed through the mountain channels, joined and inundated a river, and moved through a hydroelectric generating plant and a neighboring hamlet just off the stream bed. The equations of motion for these types of scenarios incorporate the moraine equilibrium, a forcing element, and a failure criterion; they ultimately yield a set of equations that resemble shallow water equations.
Figure 1. Gas extrusion and bifurcation in an oscillatory pattern for a lava dome.
Another way to think about coupling models involves a lava dome — a slow-moving dome of lava infiltrated by small gas bubbles that increase in size and create larger channels of gas flow. These domes can be tens of meters thick with a lava velocity of 10
-1 meters per second. Figure 1 depicts the fairly steady gas extrusion and bifurcation in an oscillatory pattern. “Studies of magmatic degassing in domes have shown that steady gas flow can undergo a bifurcation into an oscillatory pattern with periods in the range of 10
2 to 10
3 seconds for a wide variety of volcanic settings that represent a range of lava compositions and physical parameterizations,” Pitman said. Related equations of motion include mass balance for gas and lava phases, Darcy’s law for fluid momentum balance, pressure relation for lava momentum, and an equation of state for the relationship between gas and permeability. “The equations come in two parts,” Pitman said. “There’s equations that handle the fluid flow and gas flow, and there’s equations that handle the deformation of the lava.” These sets of equations occur simultaneously.
During a cascading event, the first physical process acts as either an initial boundary condition or forcing to the second model. But regardless of whether the process is coupled via boundary conditions or forcing, researchers are interested in the composition \(y = g \circ f(x)\). “Computationally, we may or may not have large codes available that easily separate \(f\) and \(g\),” Pitman said. “In either case, simulations can be demanding and there may be issues with code availability.” He then posed a question about the best way to couple computer codes or construct surrogates for \(f\), \(g\), and the composition of \(g \circ f\).
Pitman directed attendees to previous work by Ksenia Kyzyurova, James Berger, and Robert Wolpert that compared these two scenarios. The authors were interested in determining the outcome of mapping the emulator for the \(f\) process to the emulator for the \(g\) process. The trio constructed a Gaussian process (GaSP) emulator of the composition and two separate emulators for the \(f\) and \(g\) processes. The second construction—which had smaller uncertainty bounds and more effectively represented the entirety of the data—resulted in a better emulator and superior overall output. Two separate emulators can better explore the input spaces, so this result was not surprising.
Pitman next addressed a closely related idea about physical models that are solutions to space-time partial differential equations or ordinary differential equations. The typical GaSP construction emulates scalar outputs, but researchers who are solving differential equation models invariably like to emulate output fields in space and/or time. Can the coupling of space-time fields happen efficiently, and can deep learning approaches aid in emulator construction? In a recent paper, Annie Sauer, Robert Gramacy, and David Higdon developed a fast three-layer GaSP to investigate the propagation of uncertainty. Their Markov chain Monte Carlo (MCMC) method conducts a clever speedup of the MCMC convergence process.
Ultimately, Pitman presented a comprehensive synopsis of several methods for coupling physical models and accelerating the computations of coupled systems.
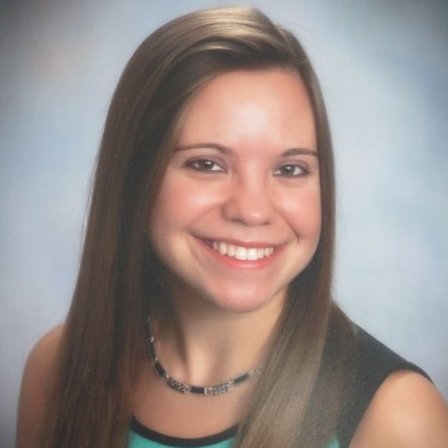 |
Lina Sorg is the managing editor of SIAM News. |