Foraging Behaviors, Predation Risk, and the Landscape of Fear
By Lina Sorg
The distribution of resource availability directly affects the locations at which animals forage for food. When engaging in productive foraging behavior, animals also need to avoid succumbing to carnivorous predators themselves; they must therefore offset these two competing interests—maximum foraging and survival—when determining how and where to optimally spend their time.
During a minisymposium presentation at the 2023 SIAM Conference on Control and Its Applications, which is currently taking place in Philadelphia, Pa., Marissa Gee of Cornell University explored the delicate balance between foraging behaviors and predation risk in food-seeking animals. “Our goal was to create an optimal foraging model that takes into account some of the richly detailed two-dimensional (2D) data that are available about 2D landscapes,” she said. Gee applies her work to Samango monkeys—which are prey for various species of eagle—and wishes to predict their spatial utilization (see Figure 1). The following motivating questions drive her research:
- Where are the optimal feeding grounds in complex spatial environments?
- What are the best paths/optimal trajectories to and from these feeding grounds?
- Can we generate meaningful usage data from simulated trajectories?
- How does food depletion in the environment affect optimal routes?
Figure 1. Samango monkeys live in Africa and travel in groups. A designated individual serves as a lookout and makes different alarm calls based on the type of nearby predator. Figure courtesy of Bernard Dupont and Flickr under the
Attribution-ShareAlike 2.0 Generic license.
Gee began with an overview of previous optimal foraging studies in the literature. Though many existing models utilize dynamic programming approaches and incorporate the impact of predators, most of these methods focus on simple discrete environments. Instead, she intends to capture the nuanced continuous data that are associated with foraging. Her work is inspired by the so-called
landscape of fear, which describes the impact of the presence of predators (real or perceived) on the foraging behavior of prey animals like Samango monkeys.
Like most optimal foraging models, Gee’s model relies on dynamic programming and treats animals as rational planners. The model system itself is a piecewise-deterministic Markov process that aims to optimize and maximize the expected utility over the fixed finite planning horizon. Animals can occupy one of three modes that represent their top priority at a given moment: (1) Foraging, (2) escaping, or (3) deceased. An animal’s specific mode can change abruptly based on the behavior of predators in their environment. The rate at which an animal can be spotted connects (1) and (2), the rate at which an animal is killed by a predator connects (2) and (3), and a final rate exists at which an animal escapes from the predator and returns to mode (1) to continue foraging.
Gee’s model exhibits standard isotropic dynamics for position. Control is the direction of motion, speed is based on both mode and location in the environment, and the energy dynamics assume a metabolic cost that is dependent on mode. Animals can only gather food in mode (1); if their energy level reaches 0 it is presumed that they have died of starvation. Gee considers three utility functions for her model: a risk-neutral linear utility, a risk-averse concave utility, and a sigmoid utility function that combines risk-averse and risk-seeking behavior. She focused on the first two utilities for the purposes of her presentation.
A coupled system of Hamilton-Jacobi-Bellman partial differential equations over three dimensions govern the models’ value functions. Gee identifies the boundary and terminal conditions and uses explicit finite differences to solve the system backwards in time for modes (1) and (2). The final reward is thus the utility of the animal’s final energy level.
Next, Gee presented a few examples of her model in action. She examined a synthetic environment with two food-rich patches, each of which is guarded by a high-risk area (i.e., a place where an animal is likely to be spotted by a predator) and contains a safe area (i.e., a den or sleeping space) in the center. The upper patch is a high-risk high-reward area, and the lower patch is a low-risk low-reward area. Gee had wondered at what point animals venture into the upper versus lower patch and wanted to investigate the level of risk that they are willing to chance in order to reach a higher reward, especially in the presence of changing levels of danger and energy.
Figure 2. Spatial image of the Samango monkey environment. Various trajectories converge around the optimal corridors that represent the best paths through which to escape a predator. Figure courtesy of Marissa Gee.
Gee explored the optimal trajectories to and from each patch. She first considered the optimal trajectory of risk-neutral planners. As their initial energy level increases, they begin to take lengthier detours to reach the food source to avoid dangerous, predator-heavy areas. But because detours on the return trip more significantly impact final reward/utility, the animals will eventually return to the most direct path in an effort to rebuild their energy. “There is lots of nuance for the optimal path to and from the feeding ground,” Gee said. She found that given the same scenario, risk-averse planners took more dramatic detours and eventually opted to remain in safe areas rather than forage as conditions became increasingly dangerous.
A second example focused specifically on the Samango environment. Gee and her collaborators generated approximate food accumulation and predator spotting rates to explore real-world optimal trajectories and transitions between modes. The various trajectories in the resulting spatial image of the environment converge around the optimal corridors that represent the best paths through which to escape a predator (see Figure 2). Gee then used trajectory data to identify high and low utilization areas for comparison with real-world examples. She discovered that their model did not closely match high utilization areas in the real-world scenario and suspects that the inclusion of safe areas might have affected the outcome. This result introduces more questions about what parameters will best capture real-world processes across the foraging and escaping modes.
Gee concluded her presentation with a brief discussion about her team’s ongoing work with food depletion, which investigates infinite versus receding horizon formulations for animals as imperfect planners.
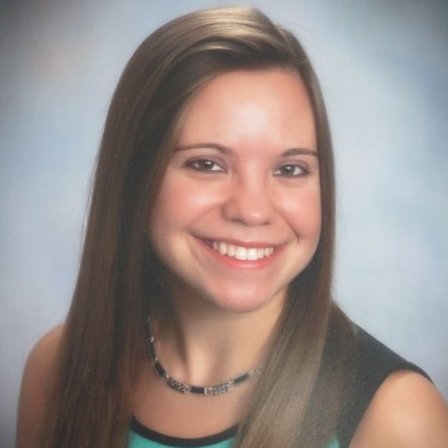
|
Lina Sorg is the managing editor of SIAM News. |