AN21 Panel Highlights Career Opportunities in Data Science
By Lina Sorg
The fields of data science and machine learning (ML) have grown tremendously in recent years, creating numerous career opportunities for applied mathematicians and computational scientists in a wide variety of industries. During the 2021 SIAM Annual Meeting, which took place virtually in July, a panel of data scientists discussed their experiences in this burgeoning field. The panel, which was sponsored by the SIAM Industry Committee, was chaired by Lalitha Venkataramanan (Schlumberger-Doll Research) and consisted of Berton A. Earnshaw (Recursion Pharmaceuticals), Stephanie Fitchett (Transamerica), Stephen Jones (The Boeing Company), and Nandi Leslie (Raytheon Technologies). After an hour of general conversation, attendees entered a series of breakout rooms to directly interact with the speakers.
Earnshaw opened the session by acknowledging the blossoming nature of ML, computer vision, deep learning, artificial intelligence, and neural networks, all of which are advancing with unprecedented speed. “It’s a fast-moving field,” he said. “A great habit to get into is setting aside time to read and stay on top of what’s going on in whatever field you’re working on. That’s a critical component for success in whatever your role ends up being.” He also suggested that researchers join a journal club or reading group to stay abreast of current developments in their particular study areas. Some companies even sponsor employee participation in such groups, though plenty of virtual meetups are readily available as well.
Leslie seconded the importance of keeping up with the latest research and encouraged attendees to take courses in programming languages to make themselves more competitive. She commented that an applied mathematics education inherently supplies many of the skills that comprise the data science toolset; for example, computational science students routinely utilize ML approaches and classic modeling techniques that involve differential equations, stochastic processes, and topological concepts. Establishing a solid background in programming and coding allows students to effectively implement these concepts and increases their hiring desirability.
Some industry employers offer regular internships that provide students with hands-on experience while they are still earning their degrees. For instance, Transamerica had three interns this past summer — all of whom were graduate students in master’s or Ph.D. programs. While some Transamerica interns come directly from data science programs, Fitchett noted that many have backgrounds in mathematics, statistics, computer science, and even physics, logic, and computational chemistry. “That’s been very fun for me,” she said.
Recursion Pharmaceuticals maintains a robust internship program and hosts three cohorts of interns every year. As the organization continues to expand, Earnshaw expects internship opportunities to grow as well. Interns typically tackle interesting problems that are carved out in convenient three- or four-month blocks, and openings are available on the company's career page. Both the BIG Math Network and SIAM's Career Resources page advertise a host of job and internship listings as well, and Venkataramanan advised listeners to join SIAM for full access to career-related information.
A panel at the 2021 SIAM Annual Meeting, which took place virtually in July, addressed the many opportunities in data science for applied mathematicians and computational scientists, and offered advice for students and early-career professionals who wish to enter the field. Top row, left to right: Lalitha Venkataramanan (Schlumberger-Doll Research), Berton Earnshaw (Recursion Pharmaceuticals), and Stephanie Fitchett (Transamerica). Bottom row, left to right: Stephen Jones (The Boeing Company) and Nandi Leslie (Raytheon Technologies).
Conversation then turned to the hiring process. While internships are certainly valuable, a lack of internship experience does not immediately disqualify applicants from job openings. In fact, Jones focuses on candidates who have applied their academic knowledge to real-world problems in any setting, including volunteer work at a nonprofit or even data analysis for graduate students in different departments. “Any experience where you’ve shown your ability to learn and applied it to a practical situation is a plus,” he said.
While it is beneficial for researchers at all career stages to work with people who are experienced in their fields of interest, this type of involvement is particularly worthwhile for recent graduates. “A big driver for the position that I was looking for was to be part of a group of statisticians who were doing the same work that I was interested in doing,” Jones said.
When it comes time to select a career path after graduation, Earnshaw urged students to refrain from dwelling too much on the typical “academia versus industry” debate and instead select the direction that feels right in the moment. Earnshaw was initially on an academic path and even held two postdoctoral appointments before ultimately pursuing industry and entrepreneurship. After completing his postdoc, he co-founded a company because it felt like a good challenge at the time. He finds his present employment at Recursion equally satisfying. “I have the opportunity to have a huge impact with my company’s mission, using the skills that I developed during school to improve the world around me by hopefully improving patients’ lives,” Earnshaw said. He also reminded attendees that the choice between academia and industry is less of an either/or decision nowadays; pursing one does not prevent participation in the other. For instance, Earnshaw is currently an adjunct professor at the University of Utah.
Earnshaw noted that the amount of on-the-job training for industry positions depends on the organization in question. For example, some larger companies have very specific onboarding experiences with mentors and rotation schedules. This process is less common for smaller companies, though many do assign mentors to bring new employees up to speed. “In a smaller company, there just aren’t as many resources to make that a formal training process,” Earnshaw said. “It probably varies very closely with the size and maturity of the company that you’re with.”
Next, panelists spoke about the balance of technical versus managerial work within the industry sector. “There are a number of opportunities in industry that are individual contributor-focused, and there are plenty of career opportunities that are management-focused,” Leslie said. “It depends on your path.” She affirmed that researchers who hope to serve as career individual contributors can spend 100 percent of their time on research and development (R&D) — even in a large, publicly traded company like Raytheon. However, one can also become an individual contributor with a business development role and work on business proposals that focus on capture management; this route requires both technical understanding of the state of the art in industry and technology as well as experience in business and management.
While some early-career professionals may choose to pursue entirely management-based careers, Leslie remarked that most data scientists begin as individual contributors. The first 10 years of a career in science, technology, engineering, and mathematics (STEM) tend to be mostly technical—at a national laboratory, they might center entirely on R&D—after which one can opt to transition to a business role.
To provide attendees with a feel for various data-science-based industry assignments, all panelists overviewed ongoing ventures at their respective companies. Fitchett is presently working on a fraud claims project that functions like an outlier detection problem and employs a model—built by two data scientists—that identifies unusual claims for accident insurance. Transamerica then sends the model to its business partners, who pick up alerts, investigate them in further detail, and occasionally forward them to the state for insurance fraud investigation.
Leslie’s current endeavors, which focus on anomaly detection in the context of cyber intrusion, utilize mathematical approaches that are similar to Fitchett’s methods. Because anomaly detection is a broad field, its techniques are applicable to multiple different areas.
In contrast, Jones is tackling a regression-like problem to understand the quality of a particular aircraft structure. His team created a sampling plan to examine the aircraft and developed a model to understand quality defects in the production system. The group then feeds the analysis to the engineering team, which ensures that the product can tolerate any quality issues that may arise. In this case, data science and statistical analysis comprise small parts of a larger project on the assembly of aircraft structure.
Earnshaw then discussed his work at Recursion, where researchers generate large amounts of data about several types of cells under different conditions that mimic diseases. They use fluorescent microscopy to compare images of healthy cells, diseased cells, and diseased cells after the application of various drugs. “One primary data science and ML task is to develop ways to extract the relevant information from images in a way that makes sense when compared with all other possible representations of all other kinds of cells and diseases,” Earnshaw said. After doing so, the team can answer questions about the cells’ appearances and assemble a statistical method for the drugs and concentrations that might potentially treat certain diseases.
Despite the popularity of data science and ML, the speakers agreed that most companies still utilize conventional procedures in some capacity. Leslie noted that researchers frequently employ closed-form traditional approaches for comparison purposes or big-picture analyses. “ML is definitely getting a lot more of our attention, but it depends on the industry,” she said. “People are still looking to graph theory and game theory, and many other approaches are still used.”
Nevertheless, data science and ML’s influence on modern-day society is substantial. Researchers now work on a number of problems that were not feasible before novel procedures like the data-driven and imperial-style approaches that accompany successful applications of ML. Biology is a prime example of a field that has profited immensely from data science because its complexity prevents closed-form solutions. “A lot of the techniques that I learned as an applied mathematician would not even work here,” Earnshaw said, since many traditional applied mathematics tools cannot effectively handle the data’s size.
Applied mathematicians, computational scientists, and other members of the STEM community will continue to benefit from the career opportunities that are associated with the growth of data science and related fields. Given the ever-increasing amounts of data in nearly every aspect of society, researchers who embrace data science practices and keep up with the state of the art will remain highly qualified for a multitude of industry positions.
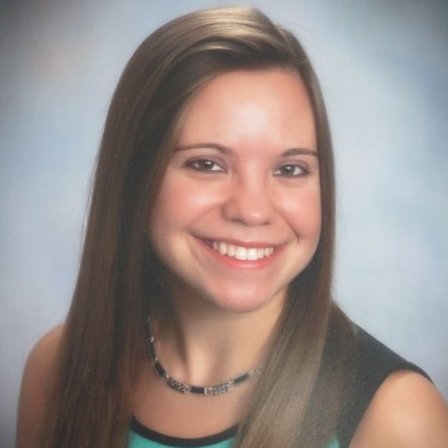 |
Lina Sorg is the managing editor of SIAM News. |