AI4Seismic Platform Combines Artificial Intelligence and Seismic Data Analysis
By Lina Sorg
The continued evolution of artificial intelligence (AI) is deeply affecting many fields of science and mathematics and will continue to do so in the coming years. Present AI techniques fall into the category of “narrow AI”— agents that utilize sophisticated technology to handle one particular task on a specific domain. Such tasks include facial recognition, language translation, and object detection. Broad AI, which is a large step above narrow AI in terms of complexity, involves multiple tasks that exist across several domains. And general AI—the hypothetical ability of a system with progressive computing abilities to handle any generalized task with human understanding—is a future goal for the field. Society is currently hovering somewhere between narrow AI and broad AI, and actively working towards the latter (see Figure 1). “We’re investigating ways to transfer some of the knowledge that we’ve obtained to other domains and applications,” Renan Souza of IBM Corporation said.
Figure 1.The evolution of artificial intelligence (AI).
During a minisymposium talk at the
2021 SIAM Conference on Computational Science and Engineering, which is taking place virtually this week, Souza introduced AI4Seismic: an AI-driven platform to accelerate geological discoveries in the oil and gas industry. “The main idea here is to allow us to capture domain-specific knowledge from geologists and represent it in a formal way that machines can understand,” Souza said. “We need to understand how geologists develop their work so that we can capture this information and do the right work for their pain points.”
IBM’s efforts to advance AI relies on three main pillars: AI Technologies for Vision and Data-driven Models, Fit-for-purpose Knowledge Engineering, and Practice and Context Understanding. All three categories capture various aspects of seismic data. The first pillar comprises deep learning (DL) for seismic image understanding, machine learning (ML) for geospatial data and reservoir analogues, data-driven well log analytics, and AI for model blending. The second pillar embodies the construction, adaptation, and evolution of domain ontologies; improved interpretability and causal analysis; training with small data; data augmentation for ML; and natural language knowledge extraction and representation. And the final pillar includes contextual and practice knowledge capture, cognitive interfaces, and end-to-end AI solutions lifecycle support for continuous learning.
The interpretation of seismic data is extremely significant in the oil and gas industry since this data provides information about strata configuration and structural settings (see Figure 2). The use of AI in conjunction with seismic data might be able to glean additional information. Seismic surveys—which are common in oil and gas exploration and utilize reflected sound waves to produce scans of the Earth’s subsurface— involves long, complex processing chains. “Most tasks are knowledge intensive, so the geologists need to understand very particular parts of their images,” Souza said. Because of its massive size, a single survey can contain tens of terabytes. All of these factors create a huge opportunity for the application of AI.
Figure 2. Seismic data provides information about strata configuration and structural settings.
Furthermore, many oil companies are currently digitalizing their data. The constant increase in the size of data sets routinely overwhelms human processing capabilities, therefore burdening geologists and increasing overall demand for data scientists. AI should thus empower the experts and accelerate the seismic interpretation process. “We want AI to work with geologists, not replace them,” Souza said.
AI’s potential in the field of geology is vast. Possible applications include classification of seismic facies, identification of geological structures, noise detection and attenuation, pre-stack and migration processing, and velocity analysis. The use of cognitive interfaces (from the Practice and Context Understanding pillar) is meant to improve the connectivity between AI systems and geologists, especially given the vast amounts of data in question.
ML models require a combination of three components: a task (such as classification, segmentation, or clustering), an algorithm (like a convolutional neural network, decision tree, or support vector machine), and annotated training data. Different training data yield different models. In addition, the quality of seismic data is often poor since the data frequently faces problems with resolution, sample size, noise format, and even geological properties and structures. It must also be properly annotated and free to use. “The oil and gas industry is very worried about data governance, so you need to have the legal rights to see particular parts of the data,” Souza said.
To address these collective challenges, Souza and his associates at IBM set out to create an environment that enables AI research and development when applied to seismic data. The result was AI4Seismic, which encompasses ML, analytics, visual computing, knowledge representation, and reasoning. The platform covers the whole AI life cycle for seismic interpretation, ranging from data corpus to publication, workflow traceability for reproducibility, and integration with other environments. “This is very hard, as we are dealing with very specific people,” Souza said. “Computing toolboxes for [geologists] is kind of tricky.” Such toolboxes process, annotate, visualize, and validate data.
Assembling and maintaining the seismic data corpus involves many steps. One must gather, curate, and annotate the seismic data; collect regional information; validate the results; and manage the synthetic data models. “We are developing several algorithms to apply visual computing and DL techniques to create an image structuring machinery to aid experts with decisions that involve geological characterization based on seismic data and domain knowledge,” Souza said. The team has also produced several web-based tools.
Souza demonstrated AI4Seismic with a video tutorial about a study of salt domes that walked viewers through the interface and revealed its numerous features. By developing state-of-the-art ML algorithms and applying them to geological situations, Souza and his colleagues created an integrated platform that uses AI-based tools to analyze seismic data and address complex problems in the oil and gas industry.
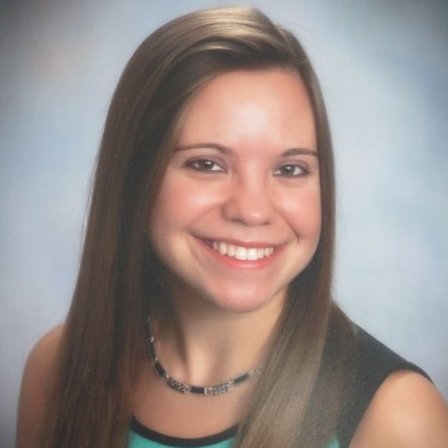 |
Lina Sorg is the managing editor of SIAM News. |