Agent-based Models Shed Light on Blue Whale Migration
By Lina Sorg
Blue whales are the largest animals ever known to have lived on Earth. Adult blue whales are typically between 80 and 100 feet long and can weigh as much as 200 tons. Despite their size, these marine mammals feed exclusively on krill—a small, shrimp-like crustacean—and can consume between four and eight tons of food per day. Their reliance on krill makes them a highly migratory species.
Northern Pacific blue whales migrate up and down the California coast in search of krill. From January through April, they breed at southern latitudes in the Gulf of California. Beginning in May and extending through September, the whales migrate north to rich foraging grounds off the coast. They return to their southern breeding grounds at the end of the year. These migration patterns correlate with summer upwelling along the California coast, during which cool, nutrient-dense waters rise to the surface, causing a bloom of phytoplankton and other organisms in the lower tropic levels (see Figure 1). This bloom draws large swarms of krill and the krill attract whales and other marine mammals.
Figure 1. During upwelling, cold, nutrient-rich water rises from the depths of the ocean to the surface. This causes phytoplankton blooms. Image courtesy of NOAA Fisheries.
Previous researchers have utilized species distribution models and dynamic state models to understand whale foraging behaviors. While species distribution models have identified sea surface temperature (SST) and chlorophyll as the environmental variables most correlated with whale presence (due to phytoplankton blooms), these models do not have movement information. Dynamic state models do include movement information, but movement is limited to one dimension. “Blue whale models have given a lot of information about what is driving the whales to be where they are, but they do have some shortcomings,” Stephanie Dodson of the University of California, Davis, said. In addition to lacking two-dimensional (2D) movement data, existing models do not account for direct prey aggregates and mechanistic drivers of whale motion. During a virtual
minisymposium presentation at the
2020 SIAM Conference on Mathematics of Planet Earth, currently taking place this week, Dodson used agent-based models to better understand migration patterns in Northern Pacific blue whales.
Researchers employ agent-based models across a variety of fields to test mechanisms of underlying emergent behaviors and self-organization. Dodson creates a spatially explicit agent-based movement model for blue whales in the eastern North Pacific, where individuals are governed by a set of rules or update processes. The model explicitly includes realistic 2D movements, treats each whale as its own autonomous agent at the individual level, and can run at both the individual and population levels. Dodson examines the effects of krill and SST on migratory behaviors and is particularly interested in the way that fine-scale decisions lead to emergent properties within the whale population. By including krill in her model, she directly accounts for prey.
Dodson’s efforts couple the Regional Ocean Modeling System (ROMS) with the North Pacific Ecosystem Model for Understanding Regional Oceanography (NEMURO). Her data, which was gathered from 2000 to 2010, exhibits a 3-kilometer spatial resolution and a daily temporal resolution. ROMS models the California current, uses primitive equations for fluid flow, and is assimilative in that it utilizes current data to ensure accurate predictions. NEMURO employs a system of ordinary differential equations in each vertical column of water to biochemically model lower trophic levels. Together, these two systems produce high-quality data on krill and SST.
Figure 2. Output of the Regional Ocean Modeling System (ROMS) and the North Pacific Ecosystem Model for Understanding Regional Oceanography (NEMURO).
Dodson breaks down the output of ROMS and NEMURO into three-month increments (see Figure 2). From April-June and July-September, the results of summer upwelling are apparent in the surface krill density. She then formulates this data as a state switching model, specifically focusing on the two-state transit-forage model for the purposes of her presentation. One can classify whale movement in two distinct behavior states: transit and forage. The transit state has much larger step lengths, as the whales move relatively directly towards a new destination. Forage behavior sees much smaller step sizes because whales cover less ground and frequently rotate in search of their prey. Dodson uses her transit-forage model to explore how population behaviors emerge from individual decisions and understand how abiotic (SST) and biotic (krill) factors drive blue whale foraging behaviors. She creates a foraging probability—based on a combination of krill density and SST—to determine a whale’s current behavior state (see Figure 3).
Dodson makes several assumptions about her transit-forage model. For example, data limitations mean that the model does not account for each whale’s specific sex or history and instead simply uses an “average whale.” In addition, decisions are based only on krill and SST, future states depend only on current conditions, and whales are not tracked upon leaving the domain. For simplicity, Dodson also assumes that whales enter at the southern border between May 1 and June 1. Every six hours, the model samples conditions at the current location, selects the behavioral state, conducts an area-restricted search, chooses a movement update, and updates the location. A video of this transit-forage model is available on Dodson's website.
Figure 3. A high foraging probability does not always correlate with foraging locations of the agents.
“The transit-forage model can reproduce broad scale northward migration patterns,” Dodson said. To validate and test her model, she looked at a whale’s arrive in known hotspots and found that the model captures the northward trend in Monterey Bay fairly well. It also captured spatial differences and clustering patterns for krill in 2003 and 2008, thus confirming its ability to account for interannual prey differences. Dodson concluded by investigating the independent influences of SST and krill. To do so, she ran models with only SST or krill as inputs, then compared the results with a correlated random walk.
“The individual-based model revealed that individual decisions lead to realistic emergent properties of the population,” Dodson said. Accounting for the presence of krill was especially valuable, as the inclusion of prey is responsible for fine-scale observations at the daily, seasonal, and interannual level. In the future, Dodson hopes to incorporate satellite data into her model, understand and represent the impact of whale communication, and even include some specific whale-krill interactions. Ultimately, however, her modeling efforts reflect emergent migratory whale behavior at the population level and offer insight into the way in which Northern Pacific blue whales might respond to future changes in environmental conditions.
The contents of this presentation were based on research by Stephanie Dodson and her collaborators that was recently published in Ecological Modelling.
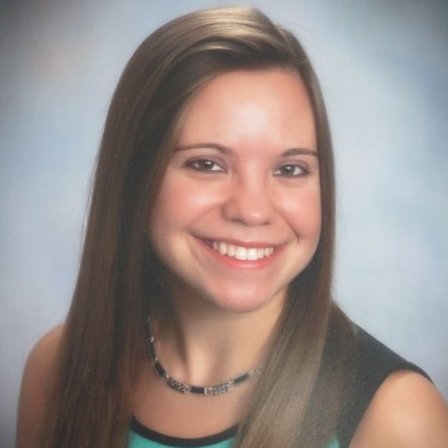 |
Lina Sorg is the managing editor of SIAM News. |