Agent-based Models Explore the Formation of Locust Hopper Bands
By Lina Sorg
Locust swarms are especially prevalent in Australia, northern Africa, and the Middle East, where they decimate crops and pose a significant threat to agriculture. In the early stage of their life cycle, locusts are not yet airborne and instead move along the ground, aggregating into coherent swarms called hopper bands. “We see these bands moving across the desert in what is clearly an example of collective behavior,” Andrew Bernoff of Harvey Mudd College said. The swarms take the form of either columnar structures or planar fronts, depending on the species and the environmental conditions (see Figure 1). During the 2021 SIAM Conference on Applications of Dynamical Systems, which is taking place virtually this week, Bernoff explored locust movement with agent-based models and their continuum approximations.
Bernoff began with an agent-based model of locusts in a columnar structure. First, he defined the model’s state space. Because they are confined to the ground, the locusts—each of which has a position and an angle of motion—move within a plane and exist in one of two possible states: a stationary state or a moving state. “It’s well known from both the lab and field studies that locusts exhibit this pause-and-go motion,” Bernoff said. They alternate between staying put or moving at a constant velocity, thus displaying a type of bimodal behavior.
Figure 1. Locust hopper bands form either a columnar structure (left) or a planar front (right).
Next, Bernoff assembled his agent-based model. He represented the locusts’ pause-and-go motion as a Markoff process and noted that if a locust is blocked from the front, it will stop moving to avoid a collision. But if a locust is crowded from behind, it will likely move forward. To account for this behavior, Bernoff and his colleagues invented a so-called blocking score. “Basically, when we look at a certain radius, the blocking score is increased by things that are in front of us and decreased by things that are behind us,” he said. “The transition rates are exponentially related to this blocking score.”
Bernoff then addressed locust orientation. The insects’ angles change according to four torques: alignment with each other, the environment, attraction/repulsion, and noise. Orientation is perhaps the most important, and Bernoff accounts for it by drawing a radius around the focal locust and aligning it with a weighted average, thus exhibiting a Kuramoto-type coupling that allows for some nice analysis. He also noted that locusts tend to orient themselves based on elements of their environment and frequently move in the direction of the sun. Attraction/repulsion is pairwise and additive. “If I am a locust and I see something right in front of me, I turn away from it,” Bernoff said. “But if I see a locust that is far away from me, I want to be with other locusts.” Finally, he adds a type of white noise to the model.
The resulting model has 14 parameters, and Bernoff explained the significance of the parameters by separating each one from the model. He started with two different initial conditions. “You’ll notice that in this model, these two different initial conditions both form columns,” he said. “This means that we’ve probably done something right.” Doubling the density of the locusts in turn doubles the density of the columns. Bernoff then isolated the following additional factors and examined the outcomes:
- Taking out noise makes the locusts move a bit more smoothly but otherwise does not have much of an effect on the dynamics.
- Taking out environmental cues still yields a columnar structure but prevents the locusts from knowing where to go. Although the impact on each individual locust is fairly small, the collective effect is rather large.
- Taking out the pause-and-go motion does not seem to significantly impact the formation, though it does affect the individual spacing of the locusts.
- Taking out alignment—a collective sensing mechanism—causes the locusts to wander randomly and lessens their chances of moving towards an external environmental cue.
- Taking out the repulsion causes the columns to collapse into very narrow bands, indicating that repulsion is necessary to maintain bands of a particular width (see Figure 2).
- Taking out attraction prevents the locusts from forming bands altogether.
Therefore, one can deduce that both alignment and attraction are necessary to maintain column formation.
Figure 2. Removing repulsion causes the columns (left) to collapse into very narrow bands (right). The blue dots represent moving locusts and the red dots represent those that are stationary.
At this point, Bernoff switched topics and turned to the interactions between locusts and resources. His colleague measured the number of locusts in a field as a function of time by using a fixed camera overtop of a locust swarm. After observing a rapid uptake with an exponential tail, the team reproduced this behavior in a new agent-based model. Like the previous model, this model also has both a stationary and moving state; the key difference is that the locusts in this stationary state actively consume crops. Therefore, the locusts’ movement—and the speed of that movement—depend on the amount of resources. “If you have more resources, you’re more likely to stop and feed,” Bernoff said. “If you have less resources, you’re more likely to restart and keep moving.”
Bernoff homogenized the model to obtain a reaction-diffusion system and perform appropriate analysis. The rate constants depend on the resources, which decrease at a rate that is proportional to the number of stationary locusts. He then touched on the model’s unique heteroclinic connection. “It’s actually determined by the mass of the locusts and the amount of resources that are in the field,” he said. “One of the great things about this is that we have a bunch of analytical formulas that allow us to connect the state behind and the state in front.”
Although the model has 10 parameters, Bernoff can deduce some of them or measure a few directly. For example, researchers know the number of locusts and the resource density. And they can measure the velocity of the locust band, maximum locust density, the amount of resources that are left in a field after a band moves through, the length of the band from front to back, and the skewness of the exponential profiles (the sharpness of their increase/decrease). Several macroscopic observables are also available in the literature. A sensitivity analysis identified two parameters that are especially important: lambda (the rate at which the locusts feed) and delta (a combined parameter). Delta measures the ratio of the propensity of stationary locusts that begin moving to the ratio of the propensity of moving locusts that become stationary.
Bernoff looked at four million data points, graphed them in pointillist plots, and superposed the observables to identify a small parameter space that seems to reproduce the models’ macroscopic quantities. When the team compared the agent-based model and its continuum approximation to the field data, the exponential trends lined up nicely. “Not only do these reproduce each other qualitatively with the right shape, quantitatively the exponentials all fall within the right range,” Bernoff said.
Moving forward, he and his colleagues plan to utilize field data from Australia to tune the model parameters for further clarity about the morphologies and movement of hopper bands.
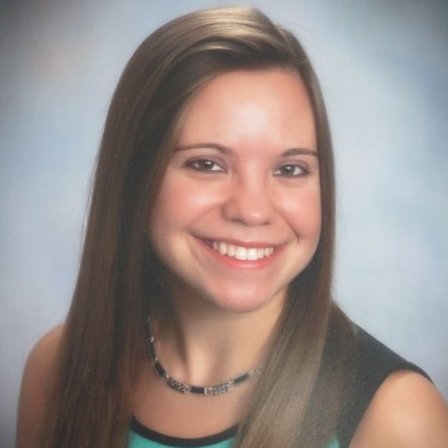 |
Lina Sorg is the managing editor of SIAM News. |